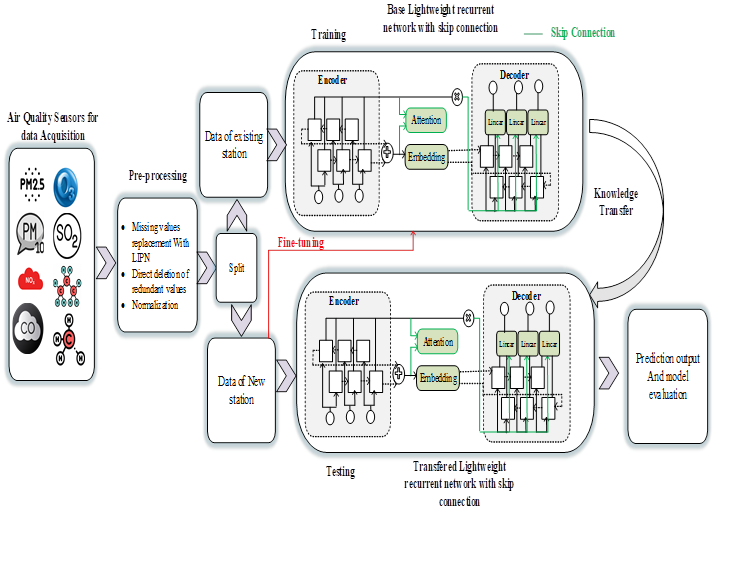
Rapid industrialization and urbanization have resulted in poor air quality, which poses a risk to human health by causing a variety of lung diseases. The precise forecast of air quality is of practical importance. Consequently, the development of an automated air pollution monitoring system based on environmental toxicology is required. Although advanced machine learning approaches can yield reasonable results in air quality prediction, they require more historical data collection. In order to address this problem, a lightweight recurrent network based on transfer learning with skip connection (LRN-SC) is proposed for air quality prediction. LRN-SC pretrains the model using data from an available station. The features that were learned from the previous station are retained, and the pre-trained model is then adjusted to fit the new one. After that, Transfer learning-based light weight recurrent network with skip connection (TL2RN-SC) is trained, and the model is tested using data from the new station. The proposed model reduces the decoding burden by adding skip contacts between the decoder and the linear forecasting layer. The simulation results show that the proposed model outperforms the existing models by attaining average RMSE and MAE of 0.974 and 2.63 respectively.