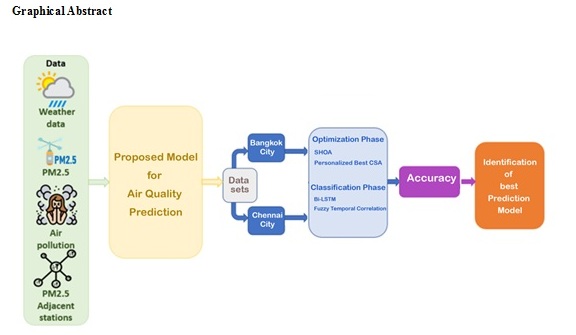
Recently, the environmental pollution is becoming a challenging issue due to the lacking of awareness about the importance of the environment and the growth of transportation facility and the various factories. The Internet of Things (IoT) technology is useful today for collecting and updating the data dynamically from anywhere anytime in less expense. Air quality of different cities is predicted by considering and maintaining the above listed technique which is useful for identifying and predicting the air quality and measure the air pollution level as well. This paper proposes a new IoT aware air quality prediction system that incorporates the newly proposed classifier with fuzzy temporal correlation and the auto-encoded Bidirectional Long Short-Term Memory (Bi-LSTM) to predict the diseases such as heart, cancer and diabetes. Moreover, we propose a new optimization algorithm called Spotted Hyena and Personalized best Cuckoo Search Optimization Algorithm (SHPCSA) for selecting and optimizing the most contributed features that are helpful for enhancing the prediction accuracy with short span of time. The air quality data is collected from various cities like Chennai, Bong Kong, etc. to conduct experiments. The proposed model is proved better than standard classifier with respect to precision, recall, F1-score and accuracy by conducting experiments
Total file downloads: 25