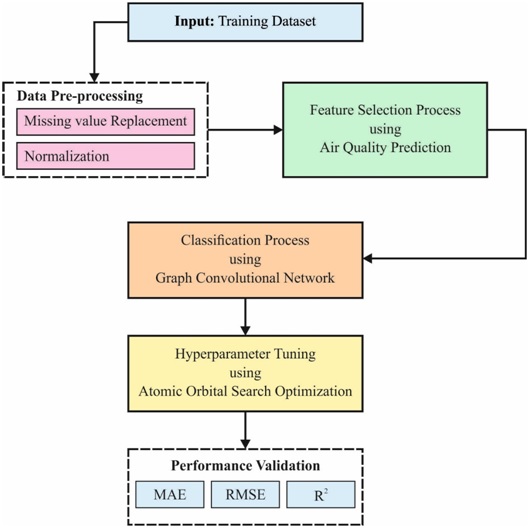
For the past few decades, owing to human activities, urbanization, and industrialization, air pollution is becoming severe across several countries. Deep Learning (DL) and Machine Learning (ML) techniques had great contribution to the development of methods in various aspects of prediction, planning, and uncertainty analysis of smart cities and urban advancement in the current scenario. Many of the cities which are developed suffered from severe air quality (AQ) because of the rapid growth in industrialization and population. In this paper, we introduce a deep learning based air pollution prediction model for smart environment design planning (DLAPP-SEDP). The presented DLAPP-SEDP technique majorly intends to predict the level of air pollution in the smart environment. It follows a three-stage process namely data pre-processing, air pollution prediction, and hyperparameter tuning. At the initial stage, the presented DLAPP-SEDP technique performs various levels of data pre-processing such as missing value replacement, categorical value encoding, normalization, and feature selection. In the next stage, the DLAPP-SEDP technique employs graph convolutional network (GCN) model. Finally, the DLAPP-SEDP technique utilizes atomic orbital search optimization (AOSO) algorithm for optimal hyperparameter tuning process, showing the novelty of the work. To demonstrate the enhanced predictive efficiency of the DLAPP-SEDP method, a wide-ranging experimental analysis can be carried out. The experimental values assured the enhancements of the DLAPP-SEDP method over other recent techniques.