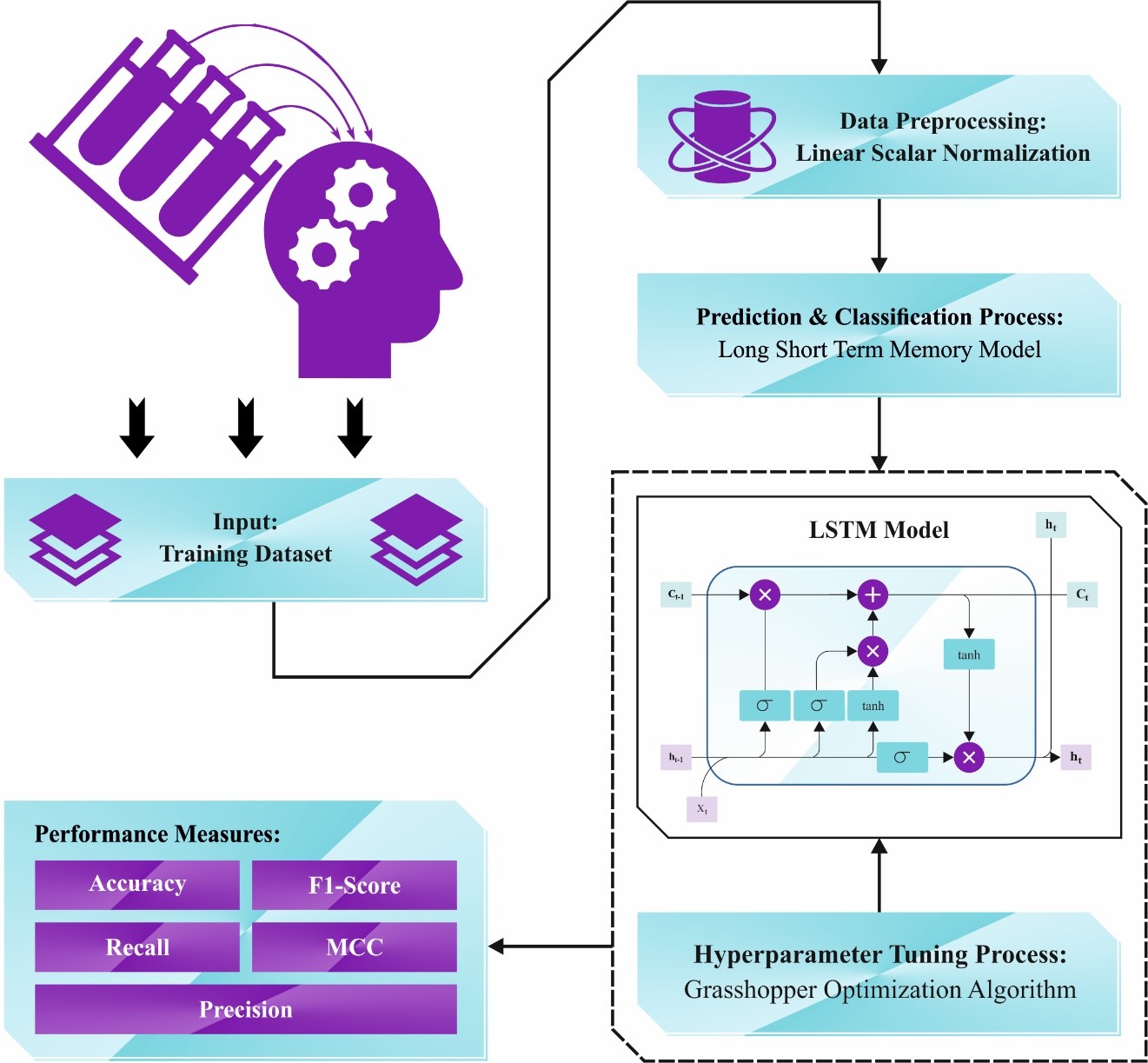
Water quality (WQ) is hugely important for animals, humans, plants, industries, and the environment. In the past few years, the WQ has been compressed by pollution and contamination. Usually, WQ is assessed utilizing costly laboratory and arithmetical processes, making real observation ineffective. Whereas, the poor WQ wants a more real and cost-effective resolution. Water pollution is a critical problem, so, it is vital to generate a method that estimates WQ in order to manage water pollution and notify users on the occasion of the recognition of poor water superiority. For effectual WQ management, it is vital to precisely estimate the WQ type. We use the advantage of machine learning (ML) models to build a model proficient in forecasting the WQ index and class. Therefore, this paper presents an automated Water Quality Index Prediction and Classification using Hyperparameter Tuned Deep Learning (WQIPC-HTDL) Approach. The purpose of the WQIPC-HTDL technique is to estimate WQI and classify the WQ into multiple levels. In the WQIPC-HTDL technique, the linear scaling normalization (LSN) approach is used. Besides, the long short-term memory (LSTM) technique is employed for the prediction and classification process. To enhance the efficacy of the LSTM model, the grasshopper optimizer algorithm (GOA) can be used. To point out the enhanced performance of the WQIPC-HTDL technique, a detailed simulation analysis was made. The obtained values inferred the rule of the WQIPC-HTDL technique when equated to other models.