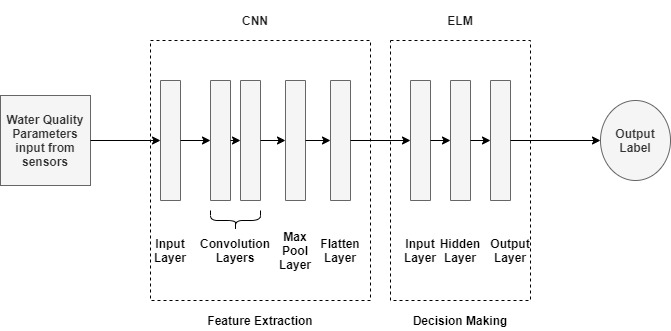
Water being a precious commodity for every person around the world needs to be quality monitored continuously for ensuring safety whilst usage. The water data collected from sensors in water plants are used for water quality assessment. The anomaly present in the water data seriously affects the performance of water quality assessment. Hence it needs to be addressed. In this regard, water data collected from sensors have been subjected to various anomaly detection approaches guided by Machine Learning (ML) and Deep Learning framework. Standard machine learning algorithms have been used extensively in water quality analysis and these algorithms in general converge quickly. Considering the fact that manual feature selection has to be done for ML algorithms, Deep Learning (DL) algorithm is proposed which involve implicit feature learning. A hybrid model is formulated that takes advantage of both and presented it is data invariant too. This novel Hybrid Convolutional Neural Network (CNN) and Extreme Learning Machine (ELM) approach is used to detect presence of anomalies in sensor collected water data. The experiment of the proposed CNN-ELM model is carried out using the publicly available dataset GECCO 2019. The findings proved that the model has improved the water quality assessment of the sensor water data collected by detecting the anomalies efficiently and achieves F1 score of 0.92. This model can be implemented in water quality assessment.