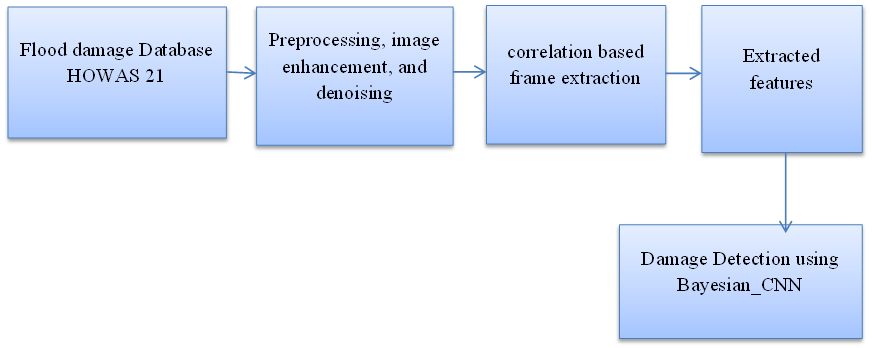
Flood management is the act of determining the frequency, magnitude, and duration of flood episodes based on the elements of a river basin that may be monitored. Floods endanger human lives and inflict significant property damage. It is essential for creating suitable flood risk management plans, lowering flood dangers, and evacuating people from flood-prone locations. Hydrodynamic methods for managing floods may be replaced with deep learning. Existing methods, however, concentrate on employing CNNs or RNNs to capture either the spatial or temporal flood patterns. Despite several advancements in flood control technology, less focus has been placed on minimising the damage that these systems do to the environment in order to boost their dependability and effectiveness. When the data is skewed, CNNs might overfit. We demonstrate how automated regularisation and uncertainty quantification allows Bayesian-CNN to get beyond these drawbacks. We have created a unique method to make use of the uncertainties that the Bayesian-CNN provides, which greatly improves performance on a big portion of the test data (around a 6% increase in accuracy on 77% of test data). By projecting the data into a low-dimensional space using a nonlinear dimensionality reduction approach, we also provide an entirely novel rationale for the uncertainty. This dimensionality reduction makes it possible to visualize the test data for interpretation and displays the data's structure in a low-dimensional feature space. This paper discusses and makes use of uncertainties for flood control while demonstrating the benefits of Bayesian-CNN over state-of-the-art technology. As a consequence, the Bayesian-CNN obtains 95.7% F1-score, 99.3% F1-score, 98.5% precision, and 98.3% recall.