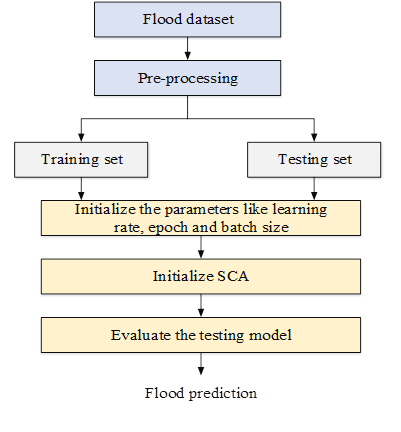
Floods inflict significant damage globally each year, underscoring the importance of accurate and timely flood prediction to mitigate property loss and life. Precise flood prediction provides governments with crucial preemptive alerts regarding potential flood disasters, enabling timely evacuations and life-saving measures. Although various ML (machine learning) models have demonstrated improved performance compared to traditional statistical models in flood prediction, they often overlook the spatial features crucial to understanding the generation of floods. DL (deep learning) is used in flood prediction to enhance the promptness and efficiency of flood levels predictions. This work presents an optimized DL model to forecast floods using time series data. Initially, the data set was cleaned and normalized by linear interpolation. Then, the DL model ODBN (optimal deep belief network) is utilized for flood forecasting time series prediction. ODBN is the integration of DBN and SCA (Sinh-Cosh algorithm). The experimental analysis is carried out on the real-time dataset and achieved better MSE and RMSE values of 0.75 and 0.94 respectively. The findings suggest that the use of an ODBN is an effective method of accurately forecasting floods.