- gnest_07250_in press.pdf
-
Paper IDgnest_07250
-
Paper statusIn press
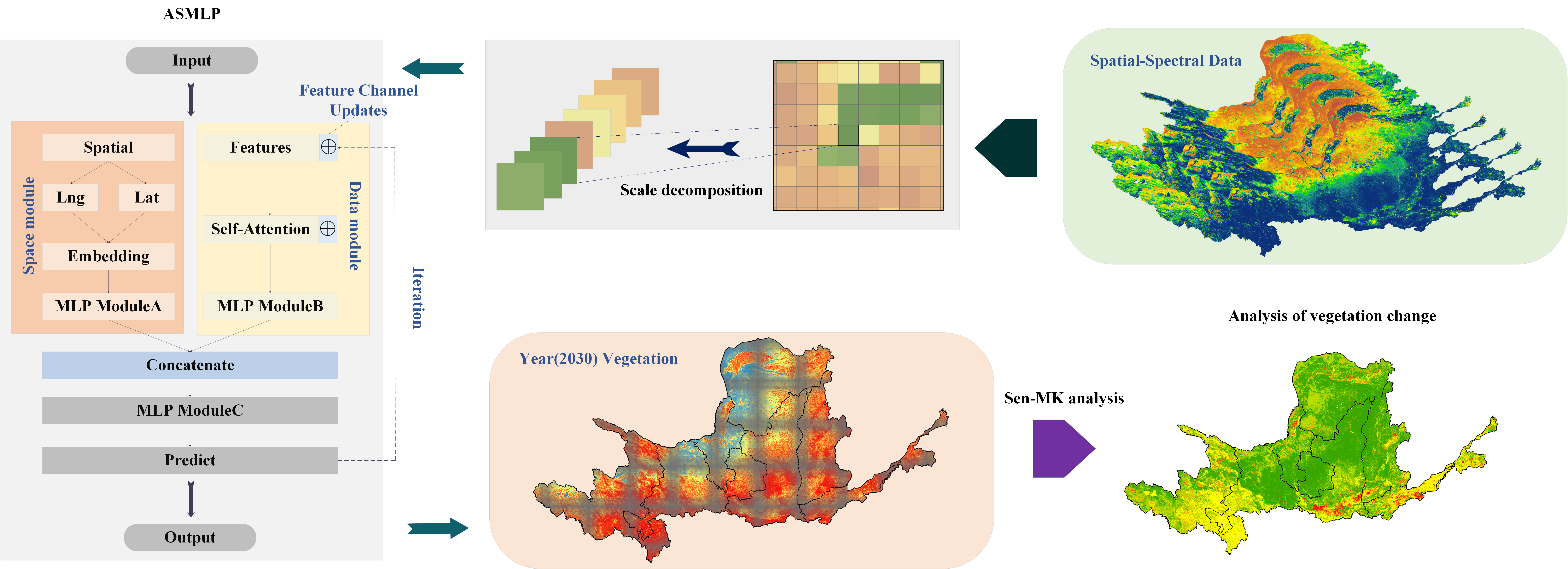
In this study, a spatial attention iterative multi-layer perceptron model (SAi-MLP) is designed based on the temporal variation characterization of the image elements based on the Spatial-Spectra data. By extracting the spatial and temporal features of the data to predict the output, the output is used as a new feature channel input to iteratively predict the value of the next time node for ensuring the temporal continuity of the time series prediction. This study was carried out using the multi-temporal vegetation content of the Yellow River Basin as the base spatial-spectral dataset. The experimental results are as follows. (1) The performance metrics of SAi-MLP compared to other models are improved by about 2% in R2 and reduced by about 0.16%, 1.16%, and 1.35% in MSE, MAE, and RMSE, respectively. (2) SAi-MLP can spatially maintain spatial nearest neighbor information compared to MLP. (3) From 2000 to 2020, the vegetation cover of the Yellow River Basin showed an increasing trend. The highest average value of vegetation cover in 2018 and 2020 is 0.78, and the average value of vegetation cover in 2030 is 0.82, an increase of 0.22 compared with 2000.