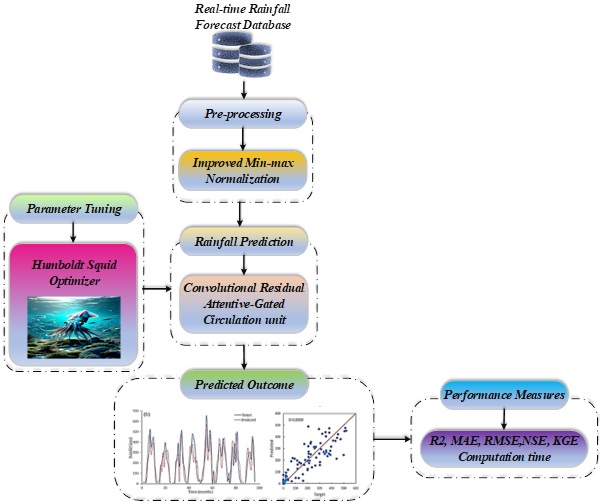
Precise long-term rainfall forecasting is becoming progressively significant, predominantly in periods of fluctuating weather circumstances. These prophecies are indispensable for several areas, including agronomy, water resource supervision, flood attentiveness, and effluence monitoring. This framework investigates the composite association between climatological data, with an emphasis on the precise estimating of rainfall by finding the effect of temperature disparities on rainfall outlines in diverse states of India. The developed framework undergoes two phases: pre-processing and rainfall forecasting. At first, the Improved min-max normalization (I-MMN) technique is highlighted in the preprocessing stage to eliminate unsolicited missing values from the database. Then, the convolutional residual attentive gated circulation unit (CResAtt-GCU) technique is presented to envisage the amount of rainfall that occurs in various Indian states. Finally, the Humboldt squid optimizer (HBSO) technique is presented to tune the parameters of the developed model to minimize network complications during the training process. The developed framework is processed under the Python platform and the real-time rainfall prediction database collected between 2000-2023 is utilized for the experimentation process. Various computational measures like R2, root mean square error (RMSE), Kling-Gupta Efficiency (KGE), Nash-Sutcliffe Efficiency (NSE), mean absolute error (MAE), and computation time are evaluated and distinguished from different studies. The overall R2 of 0.993, RMSE of 0.84, KGE of 0.96, NSE of 0.992, MAE of 0.53, and overall CT of 86.66s are obtained by the developed technique against various conventional studies on forecasting the rainfall in different regions of India.
Total file downloads: 23