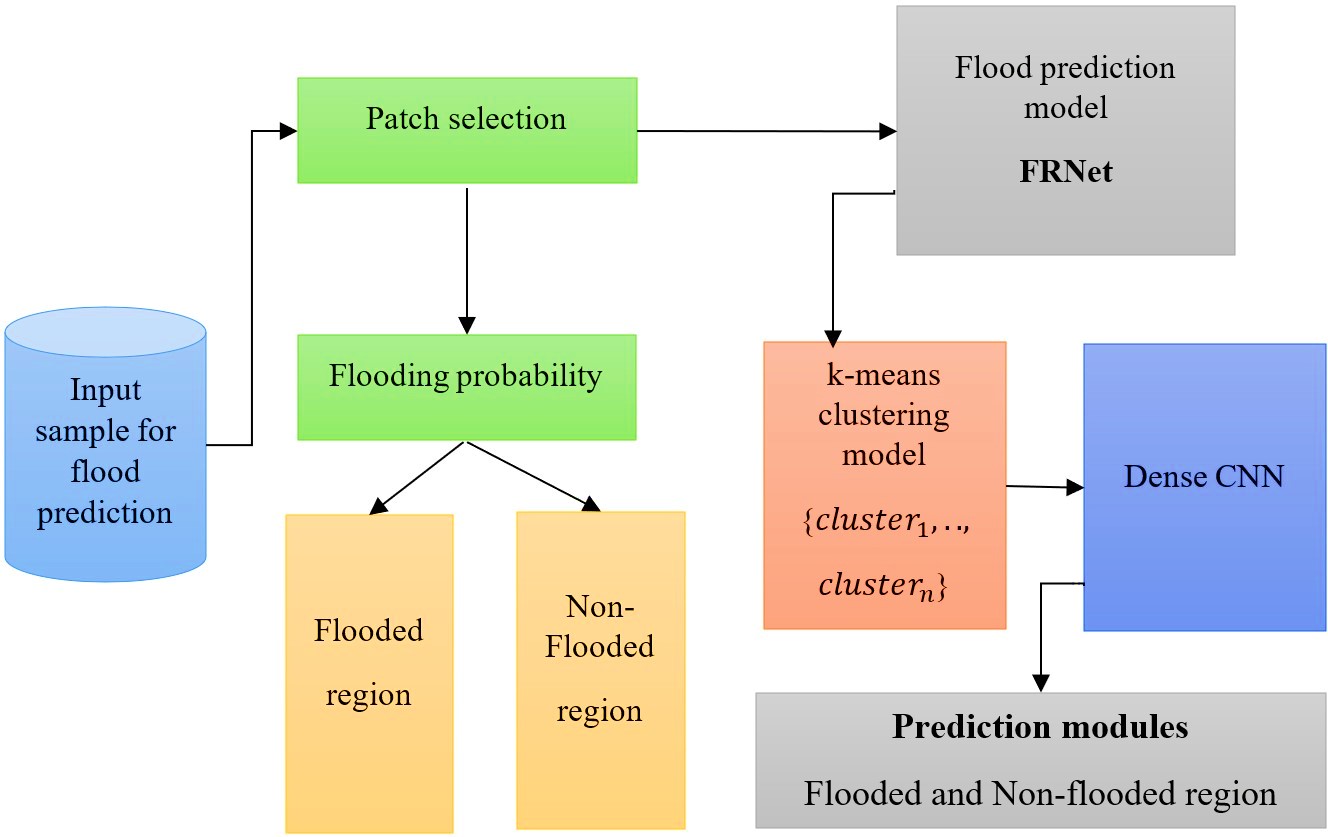
Hydrological monitoring in urban regions is crucial to analyze hydrology and manage floods.
Nevertheless, monitoring flood overflow in urban areas including the degree of flood overflow is
frequently insufficient. Hydrological models' ability to provide early flood warnings is limited by
this deficiency, making calibration difficult. This work developed a technique for assessing the
urban floods depth using deep learning and image recognition methodologies to overcome this
constraint. To recognize submerged things in images like a pedestrian's leg or a car's exhaust pipe,
this approach uses the object identification model known as the Flood Region-prediction network
(FRNet). The mean average precision for water depth identification in a flood images collection
was 99%. According to the research, the reference object types submerged in the flood impacts how
well FRNet detects flood depth; utilizing a car as the reference objects produced a high range of
accuracy than employing a person. Moreover, the accuracy of recognition was considerably
improved by image augmentation using learning technology. The presented method uses data as
image or video from traffic cameras that are currently in use to extract on-site, continuous, real-time
data of water depth. This technology provides an affordable and instantly deployable solution by
removing the requirement to install additional water meters.
Total file downloads: 20