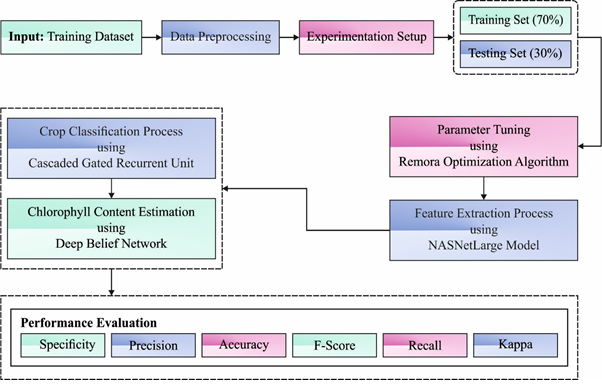
Due to the development of open access medium-high resolution remote sensing data like multispectral remote sensing images, crop classification becomes a hot research topic to be realized on large scale using machine learning (ML) models. At the same time, chlorophyll content is a critical index used for defining crop growth conditions, photosynthetic ability, and physiological position. It has an adaptive characteristic which finds useful to monitor crop growth conditions and understand the procedure of material and energy exchange among crops and the environment. Recently, several research works have been carried out to estimate chlorophyll content on multispectral remote sensing images. The recent advances in deep learning models enable us to effectively classify different crop types and estimate chlorophyll content on multispectral remote sensing images. In this view, this paper presents a new remora optimization with deep learning driven crop classification and chlorophyll content estimation (RODLD-C4E) model using multispectral remote sensing images. The proposed RODLD-C4E model intends to properly identify the crop type and chlorophyll content. For accomplishing this, the proposed RODLD-C4E model initially derives a RO algorithm with NASNetLargemodel for feature extraction process. The utilization of RO algorithm enables to effectually adjust the hyperparameters of the NasNetLarge model. Besides, cascaded gated recurrent unit (CGRU) model is employed for crop type classification. Finally, deep belief network (DBN) model is applied to estimate the chlorophyll content exist in the crop. To demonstrate the better performance of RODLD-C4E model, a wide ranging experimental analysis wasimplemented on benchmark dataset. The comparative analysis pointed out the better outcomes of the RODLD-C4E model under several aspects.
Total file downloads: 6