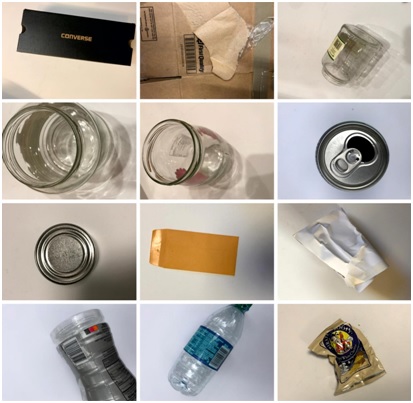
In recent days, massive quantity of waste materials gets considerable increased with increasing population. Proper management of waste materials becomes essential to reduce environmental degradation and improve quality of life in smart cities. Waste management helps to collect and treat waste materials from society. Smart waste management is a new frontier for local authorities assisting in reducing municipal solid waste and improving community recycling rate. Appropriate classification of waste objects necessitates the design of automated waste classification models based on artificial intelligence (AI) and computer vision (CV) based approaches. With this motivation, in this study, an automated artificial intelligence with earth worm optimization assisted waste management and classification (AIEWO-WMC) model is proposed for smart city environment. The proposed technique intends to recognize and categorize waste objects using the DL techniques. The proposed model primarily derives a RetinaNet based object detection module to identify the existence of waste objects in the images. To improve the classification performance, Adagrad optimizer is applied. Moreover, earthworm optimization with stacked autoencoder (SAE) algorithm is applied for the classification of waste objects. To assuring the improvised results of the AIEWO-WMC technique, comprehensive experimentation is performed on standard dataset and the obtained values indicated the supremacy of AIEWO-WMC model over the other techniques with increased accuracy of 99.15%.