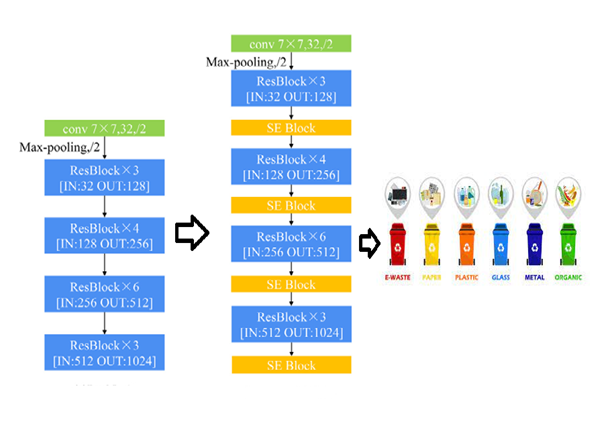
A Squeeze and Excitation Network is a deep-learning architectural component designed to enhance networks. The "squeeze" step reduces the spatial dimensions of the input feature maps, and the "excitation" step adaptively recalibrates channel-wise feature responses. This allows the network to focus on more educational features and ignore less useful ones. Garbage classification is a crucial task for sustainable environmental management. It involves categorizing waste into recyclables, organics, and non-recyclables, among other classes. Deep learning models, like the proposed Buffalo Optimizer-based SEN, can play a pivotal role in automating this classification process using computer vision techniques. Garbage Classification: There is still a long way to go until countries worldwide have successfully increased public awareness and implemented measures to prevent the rapid degradation of the natural environment. The annual global generation of e-waste is between 20 and 50 million growing components of municipal solid garbage. The disposal of electronic trash presents significant threats to environmental quality. As a result, pollution monitoring and control are crucial for maintaining a healthy ecosystem. In this research, picture-layered characteristics were extracted using an encoder composed of varying numbers of ResBlocks and the Squeeze-and-Excitation (SE) block, which was built on top of the UNet backbone network. UNet's decoder structure was streamlined, and the number of network model parameters was cut in half. In the meantime, the multiscale feature fusion module was developed to enhance the network's detection accuracy by optimizing its parameters with a bespoke loss function in place of the standard function. The African Buffalo Optimisation Algorithm is also used to fine-tune the hyper-parameters.