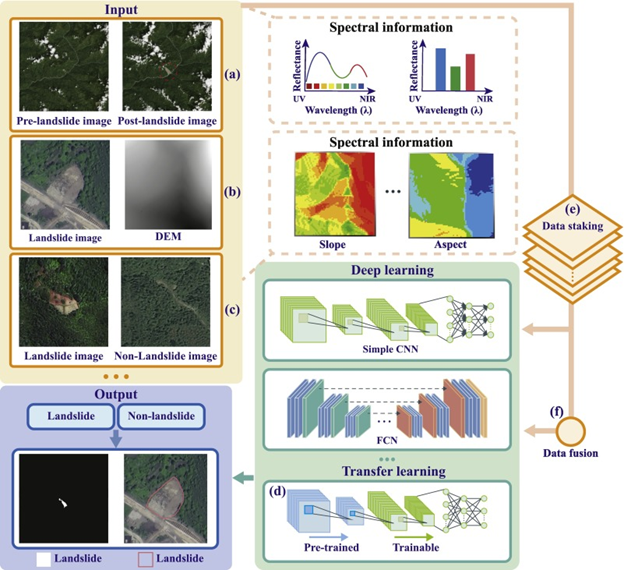
The precise analysis of conditions in the landscape before and aftermath of the disaster is a mandatory challenge in aerial image landscape monitoring. The change in patterns of landscape, damaged pathways, and damaged areas will have a major impact without monitoring and redevelopment. Therefore, semantic segmentation of the landscape is required in order to analyze the changes and avoid other risks in pre-and post-disaster scenarios. To address these queries a deep learning-based landscape monitoring method is presented in this work. A Gated Shaped Convolution Neural Network is utilized for the semantic segmentation of the aerial landscape images. Initially the aerial image undergoes pre-processing with the process of dilation and GSCNN emphasize the shaped and boundary masks of the affected landscape. To choose the best possible pathways and solutions for development the GSCNN undergoes the particle swarm optimization. In the present study, the proposed PSO-GSCNN is evaluated by comparing the accuracy, precision, and recall of the proposed method with Restricted Boltzmann Machines (RBMs), Convolutional Neural Networks, and Fuse-Net segmentation methods. In comparison with conventional RSM, CNN, and Fuse-Net, the accuracy rate of the model is 97.65%, the precision rate is 98.21%, and the recall rate is 97.23%. This technique has achieved 97.66% accuracy, 97% precision, and 96% recall, all higher than the existing methods.
Total file downloads: 5