You are here
Prediction intervals for carbon dioxide emissions in china by extreme learning machine ensemble based on particle swarm optimization
Pages :
1 - 9
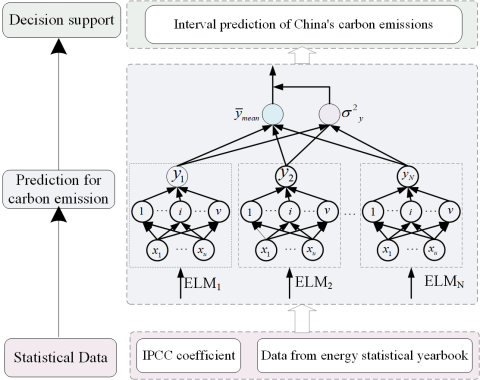
The emission of carbon dioxide is the major cause of the greenhouse effect, which has a negative impact on human survival and sustainable economic development; therefore, it is very significant for discovering the underlying influential factors of carbon dioxide emissions. In this paper, an extreme learning machine ensemble based on particle swarm optimization approach(PSO-ELMEnsemble) are applied to predict the emission of carbon dioxide, which provide estimated values as well as the corresponding reliability. In addition, the particle swarm optimization approach is used to optimize the connection weights of extreme learning machine. The performance of the proposed PSO-ELMEnsemble is experimentally validated by carbon dioxide emissions data during the period 1978-2016 by using state-of-the-art comparative method, where the proposed approach outperforms the others in achieving the best trade-off between accuracy and simplicity.