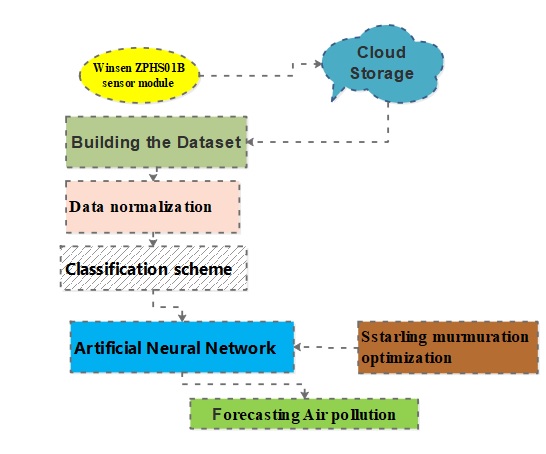
Air pollution monitoring is becoming increasingly important, with an emphasis on the effects on human health. Because nitrogen dioxide (NO2) and sulphur dioxide (SO2) are the principal pollutants, many models for forecasting their potential harm have been created. Nonetheless, making precise predictions is nearly impossible. The prediction of air pollution enables researchers to understand how pollution affects human health. Deteriorating air quality can lead to respiratory diseases such as lung cancer and asthma. The effect of pollution on environmental degradation can also be predicted and reductions can be detected in the ozone layer. This study also focuses on and promotes the development of smart city environments by obtaining influential pollutants that affect the air, thereby reducing the source of specific pollutants An Artificial Neural Network (ANN) model is used as a forecast the pollution and the starling murmuration optimization (SMO) procedure is used to optimise the Artificial Neural Network strictures to achieve a lower forecasting error. Furthermore, in this research work, we used real time dataset as we have used Winsen ZPHS01B sensor module to collect the data, which is stored in cloud platform. After the composed data is used to train and test, after this process we will evaluate the results. To assess the performance of the suggested model. Furthermore, the perfect has been tested using two alternative kinds of input parameters: type as, which contains various lagged values of variables (NO2 and SO2), and type as, which only includes lagged values of the yield variables. The collected findings suggest that the projected model is more precise than existing joint forecasting benchmark models when different network input variables are considered.