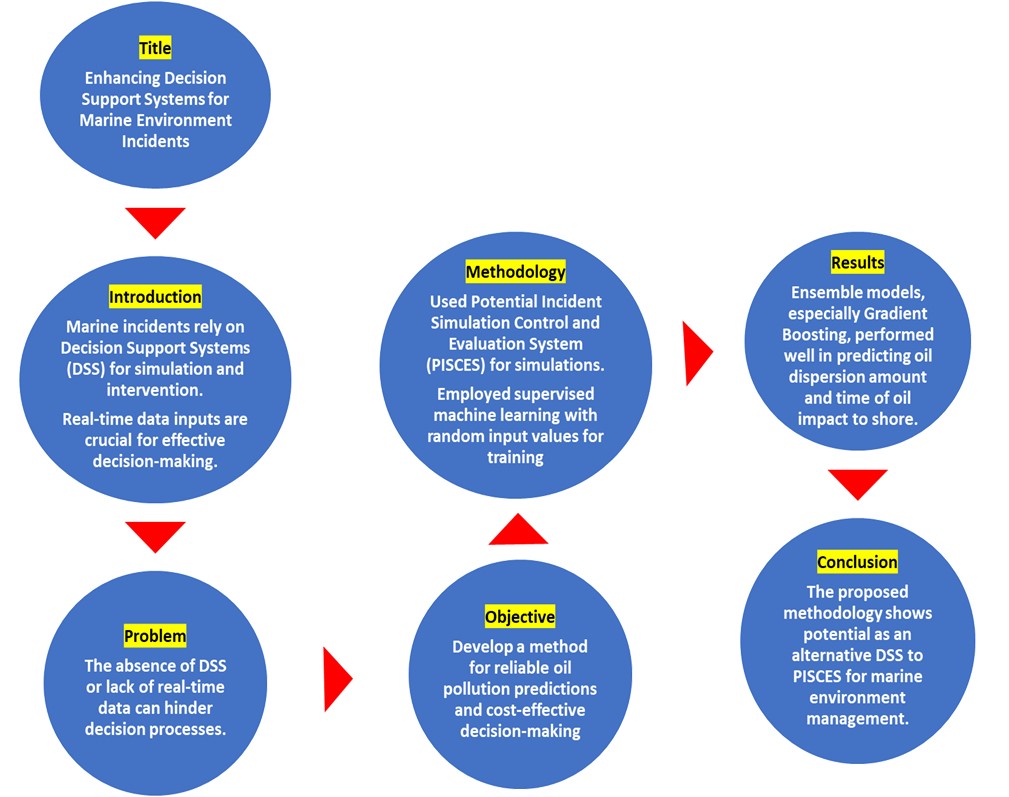
The incidents occurring within the marine environment are supported by various Decision Support Systems (DSS), both in simulation and intervention. Accurate and real-time data inputs into these systems greatly contribute to the effective and prompt decision-making process. However, the absence of these systems in all situations or the inability to provide real-time data inputs can negatively impact the effectiveness of decision processes. This study aims to create a method that can enable reliable and accurate predictions regarding oil pollution and the cost-effective execution of certain decision processes. For this purpose, an exploratory study with various cases of ship-sourced oil pollution has been simulated using the Potential Incident Simulation Control and Evaluation System (PISCES). Random input values for each case have been utilized in PISCES simulation experiments. Afterward, supervised machine learning models were trained using the simulation experiments data set to predict oil dispersion amount and time of oil impact to shore. Model hyperparameters were optimized using cross-validated grid-searches. Through hyperparameter optimization using grid search, XGB, Random Forest, and Gradient Boosting emerged as the leading models for estimating oil dispersion. However, while Gradient Boosting yielded satisfactory outcomes, its performance could be further enhanced with additional data. Obtained results show that the proposed methodology has the potential for predicting the time of impact to shore, hence for rapid results for standard initial actions, they can be used as an alternative DSS to PISCES.
Total file downloads: 7