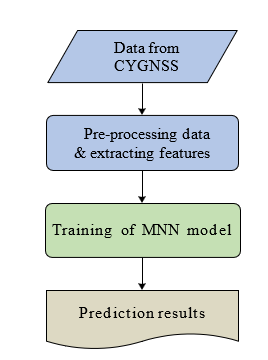
Recently, there has been a rise in research based on data-driven based machine learning models to address the issue of processing speed posed by various physiological simulations. The majority of studies, however, have concentrated on creating models for particular water sources, drainage systems, and gauge stations area. Therefore, unless further data is supplied and the models are subsequently trained, their outcomes cannot be effectively applied to different scenarios. With its excellent spatio-temporal accuracy range with the advanced analytics, the forthcoming Global Navigations Satellite Systems (GNSSs) technology offers a novel method for the monitoring of flood disasters in dynamic manner. This study recommends a Multi-layered Neural Network (MNN) for flood monitoring, which combines a Convolutional Neural Networking (CNN) along with a backward propagation based (BP) learning network. The CNN component is used to obtain the abstracted features from full delay doppler maping images. The BP section is provided with GNSSs characteristics, such as reflection of surface with power range, and also the vegetation attributes from the Soil Moistening Active Passives (SMAP) satellite source data. In comparison to physiologically based models, the results shown that the CNN-oriented system makes accurate predictions on "unseen" areas of catchment with a substantially shorter processing time. The obtained results imply that, with respect to accuracy of prediction, the patch-enabled option is superior to the resizing-based option. Additionally, every experiment has demonstrated that the forecast of flow speed is more precise than the prognosis of water depth, indicating that the water deposition is more complex to information about global level elevations than the flow speed with velocity.