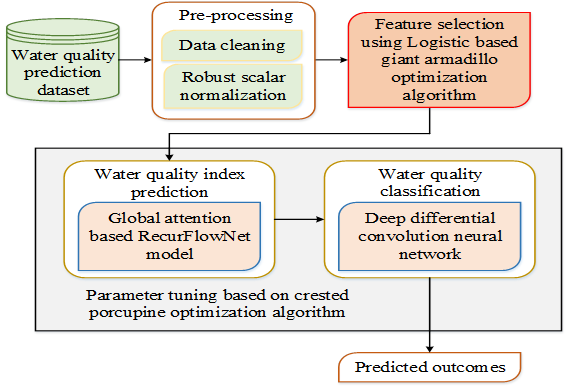
Water quality prediction and classification plays a crucial role in ecosystem sustainability, agriculture, aquaculture and environmental monitoring. The nonlinearity and nonstationarity of water quality are challenging for traditional prediction techniques to adequately capture. The rapid advancement of deep learning in recent decades has made it a hot topic for predicting water quality and classification. In this paper, a new Optimization driven Deep Differential RecurFlowNet (ODD-RecurFlowNet) model with feature selection is proposed for predicting and categorizing the water quality. Preprocessing methods are utilized to evaluate the collected data to predict the water quality class and water quality index. Before deploying feature selection algorithm, preprocessing procedures such as data cleaning and robust scalar normalization are carried out. A logistic based giant armadillo optimization algorithm (GArO) algorithm is used for optimal feature selection. Next, the water quality index is predicted using global attention (GA) based RecurFlowNet model. Subsequently, a deep differential convolution neural network (DDiff-CNN) model is employed for the classification of different levels of water quality. In addition, the hyper-parameters of ODD-RecurFlowNet is tuned using the crested porcupine optimization algorithm (CPoOA). For simulation, python platform is used and the standard water quality dataset from Kaggle library is used to validate the experiment. The finding shows that the proposed ODD-RecurFlowNet model obtains the overall accuracy of 98.01% and RMSE value of 0.039. Thus, the obtained results prove the superiority of proposed model to the existing methods.
Total file downloads: 31