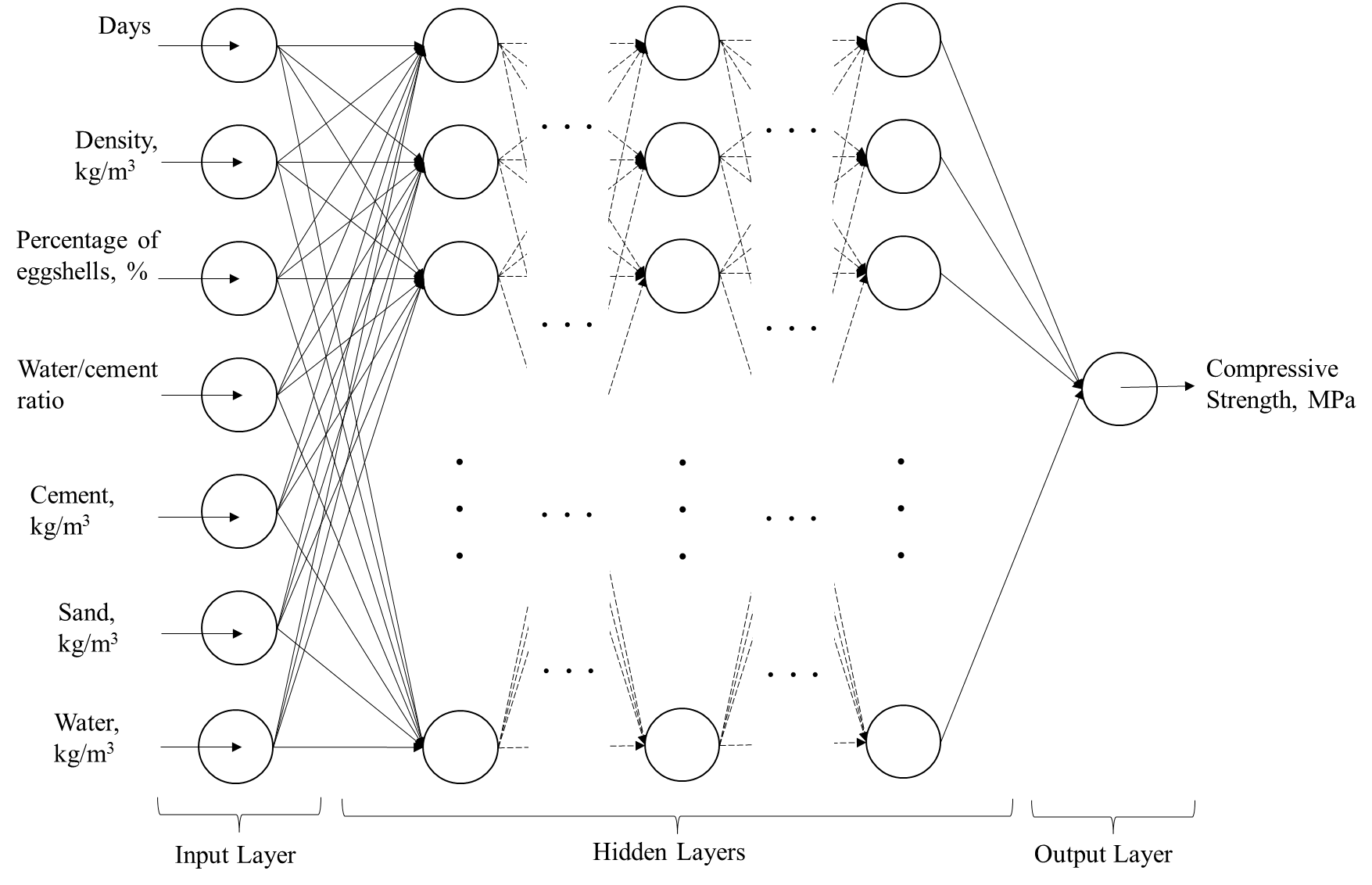
The use of eggshell-based foamed concrete represents a sustainable approach to enhancing environmental friendliness in construction materials. This study investigates the predictive modelling of the compressive strength of eggshell-based foamed concrete through a deep learning model, fine-tuned via Bayesian optimization. Utilizing a dataset of 360 samples with diverse input parameters, the model was optimized with four hidden layers (28, 21, 28, and 21 neurons) and the Rectified Linear Unit (ReLU) activation function. The model demonstrated excellent predictive accuracy, achieving a mean squared error of 0.0522, a mean absolute error of 0.0382, and an R² value of 0.9548 over 200 epochs. Notably, the water/cement ratio emerged as the most influential factor in prediction accuracy. This research provides a robust, AI-driven method for predicting the compressive strength of sustainable construction materials, contributing to advancements in environmental technology and the optimization of eco-friendly construction practices.