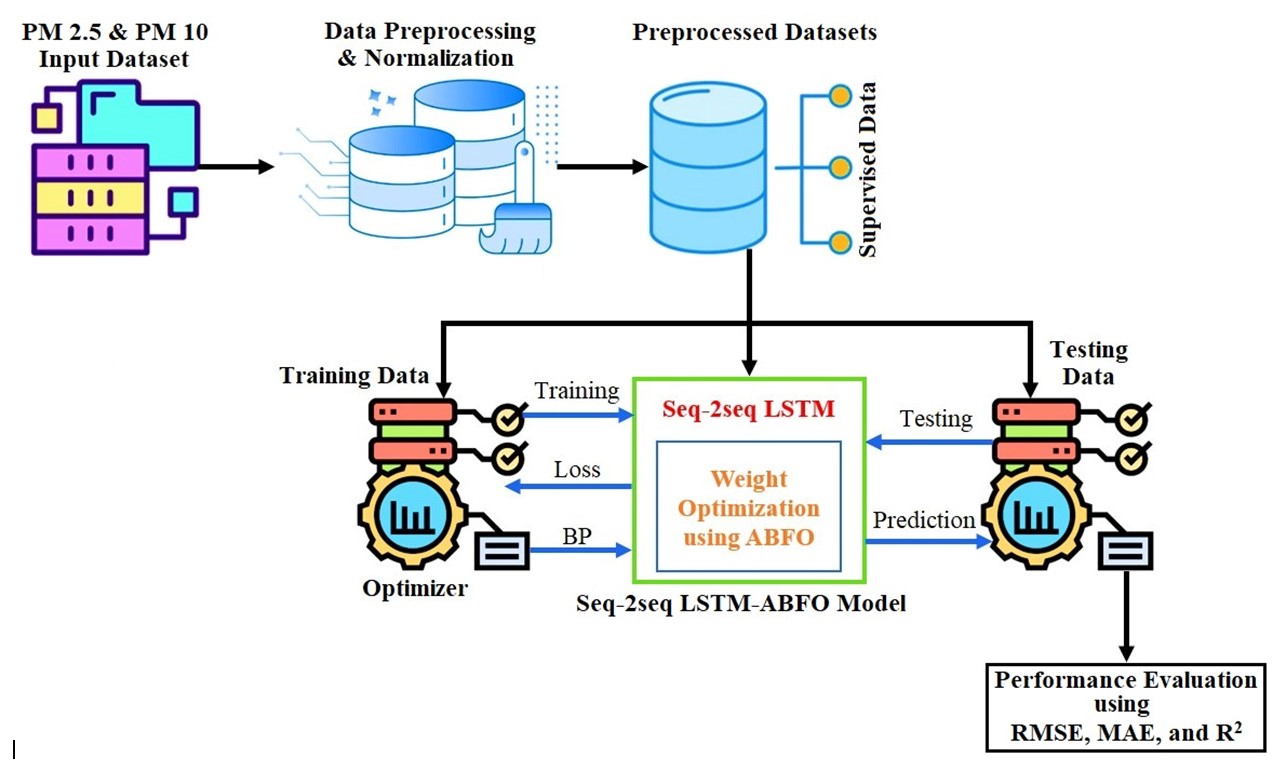
Precise and dependable forecasting of Particulate Matter 2.5 ( P M 2.5 ) and P M 10 levels hold significant importance for the public's ability to proactively mitigate exposure to air pollution and for informing governmental policy responses. Nonetheless, predicting PM2.5 and PM10 concentrations presents considerable challenges due to the complex dynamics of atmospheric flows. In existing mainstream research, most air pollution prediction models presently employ a single predictor, hence limiting the potential for enhancing stability and accuracy. This study proposes a pioneering methodology for forecasting P M 2.5 and 10 concentration levels by integrating a Modal Auto former system with the Sequential-to-Sequential predictive model. The Seq-2Seq network model leverages sequential learning and square transformation of Long Short-Term Memory (LSTM) techniques for improved accuracy in P M 2.5 and 10 concentration prediction. Additionally, the incorporation of a Modal Auto former enhances the predictive capabilities by efficiently capturing nuanced variations in atmospheric conditions. Proposed Seq-2Seq LSTM network predictor is given a weight, and the Adaptive Beetle Feelers Optimization (ABFO) algorithm is utilized for weight optimization to attain the best prediction results. Through rigorous experimentation and validation, proposed approach demonstrates superior performance compared to traditional methods using Air Quality Data in India from kaggle, offering a promising avenue for precise P M 2.5 and 10 concentration forecasting with practical implications for air quality management and public health initiatives.