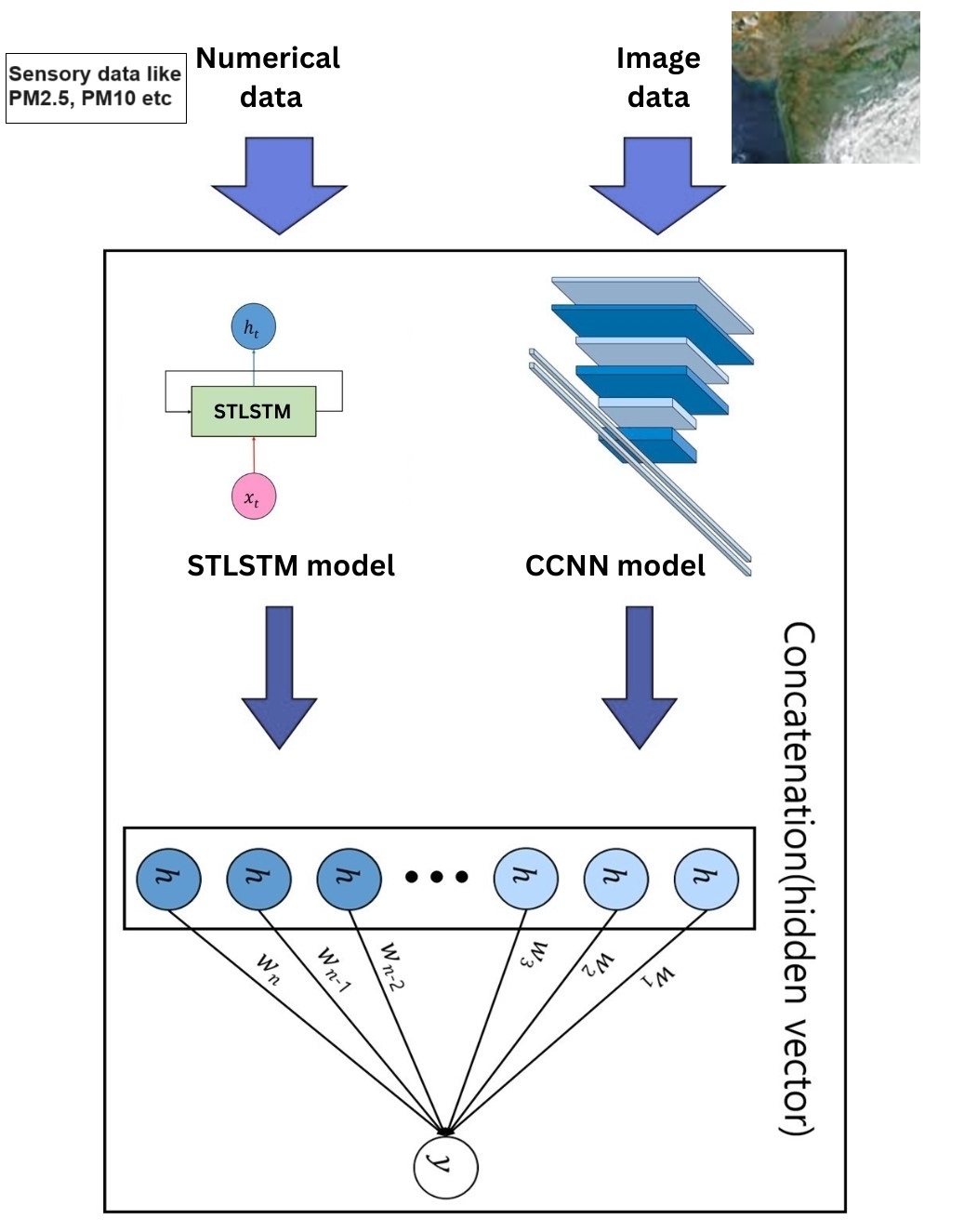
With the rapid expansion of science and technology, there is a growing hazard to public health from many forms of pollution in the air, particularly fine dust, which can aggravate or induce heart and lung disorders. Furthermore, this threat is expanding as a consequence of the rapid progression of technology. The purpose of this study is to make an attempt to forecast the fine dust concentration in Delhi eight hours in advance in order to reduce the potential adverse impacts on health. The objective of this study is to develop a multimodal deep learning framework that combines the architectures of Self Tuned Long Short Term Memory (STLSTM) and Concatenated Convolutional Neural Network (CCNN) in order to generate accurate predictions. This research is constructed using a dataset that contains both numerical and visual data. An STLSTM AutoEncoder is responsible for handling numerical time series data, in contrast to the Concatenated Visual Geometry Group Neural Network (CVGGNet) models (CVGG16 and CVGG19), which use image data to compare performance depending on network depth. Based on the results of the final investigation, it has been predicted that the deeper CVGG19 model performs up to 14.2% improved than modality models with single data input that simply use numerical data. The RMSE, MAE, SMAP of the proposed model is 3.87, 3.45, 09.87 respectively. When compared to the models with single data input, the multimodal deep learning model that makes use of both types of data performs significantly better.