-
Thematic area
-
VolumeVolume 27 (2025)
-
Issue
-
Pages1-7
- gnest_06802_final.pdf
-
Paper IDgnest_06802
-
Paper statusPublished
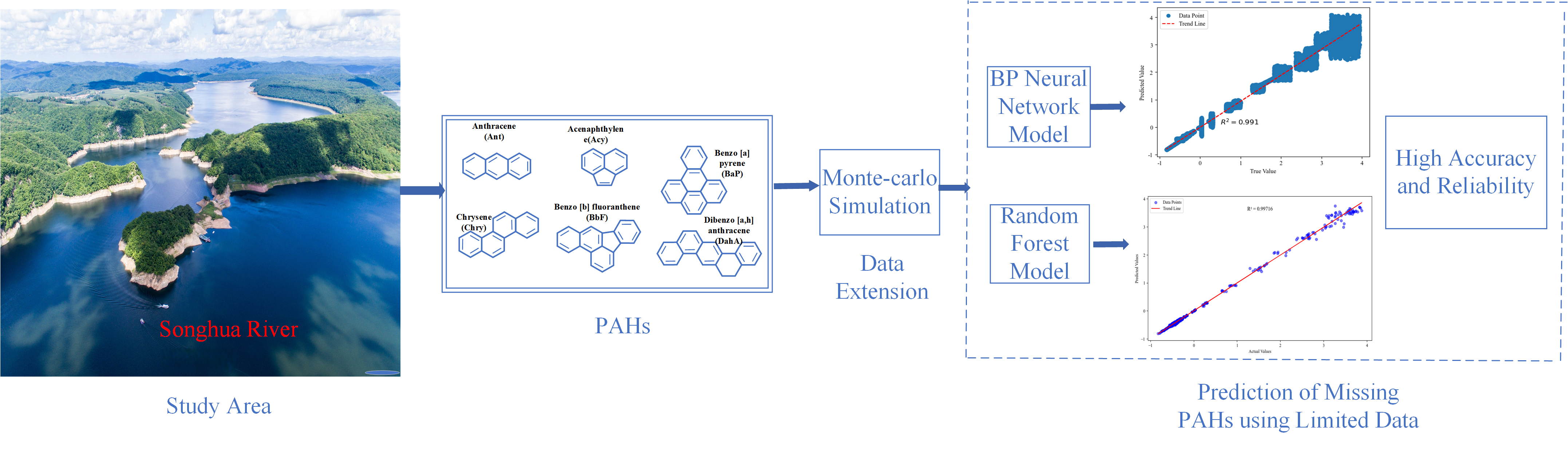
Polycyclic aromatic hydrocarbons (PAHs) are toxic and ubiquitous in the water environment. The content of PAHs needs to be systematically and comprehensively detected to achieve the environmental quality assessment. However, due to the constraint of testing cost, detection data in the Songhua river are often missing in polluted sites. In this study, the contents of 6 PAHs in the Songhua River were predicted by utilizing limited data. Data were expanded by Monte Carlo, and BP neural network and random forest models were built to predict the contents of PAHs in missing samples. Both models showed good training effects, with high accuracy and reliability. BP neural network was slightly better than random forest in prediction of the contents of PAHs based on several performance indicators. However, when dealing with large amounts of data, random forest still performed well due to its inclusion of numerous decision trees. The two models could provide important decision support for the spatial analysis and evaluation of water pollution. This approach could not only help to understand the distribution patterns of pollutants in water bodies, but also improve the targeting and effectiveness of management and intervention measures.