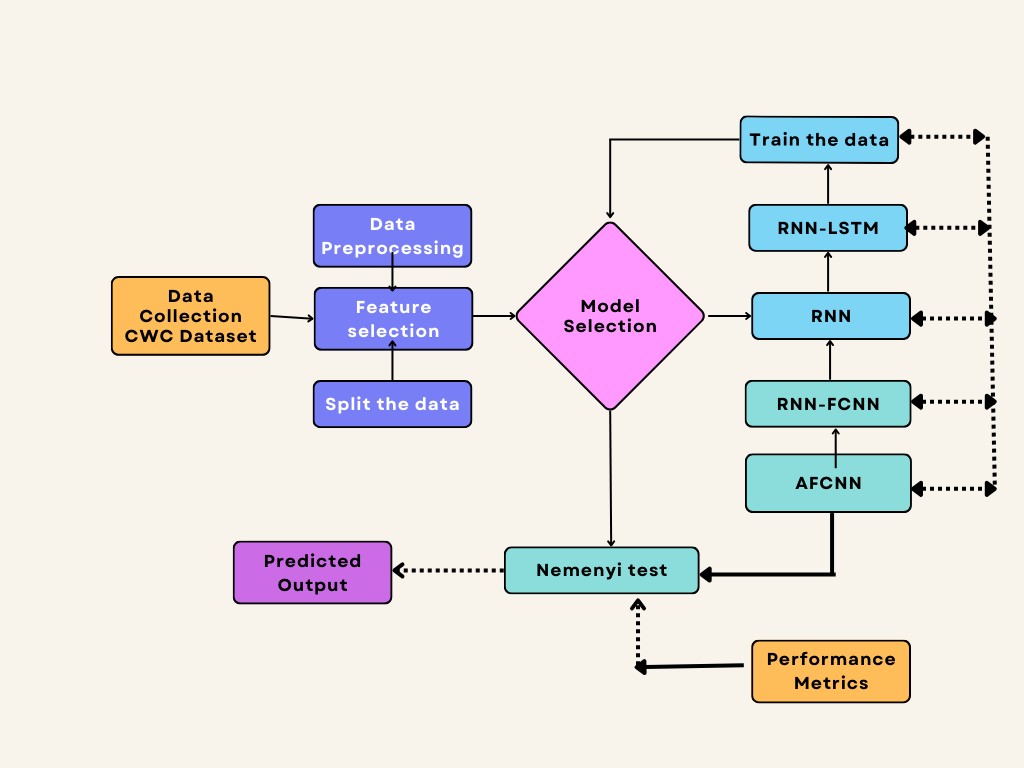
The process of sedimentation in reservoirs is an important issue that must be addressed for effective water resource management. Traditional methods for predicting sedimentation are complex and require extensive data inputs. In this study, we innovate by integrating four distinct neural network architectures into a cohesive approach for prediction. Specifically, Recurrent Neural Networks (RNN), RNN combined with Long Short-Term Memory (RNN-LSTM), RNN combined with Fully Connected Neural Networks (RNN-FCNN), and Autoencoder integrated with Fully Connected Neural Networks (A-FCNN) were utilized to estimate sediment volume based on their performance. This approach is faster, more accurate, and can handle multiple inputs. Additionally, Nemenyi and Principal Component Analysis (PCA) tests were conducted to build more robust and reliable models for estimating sediment volume. These computational methods were tested on real-world data, and the models demonstrated strong predictive performance for a specific reservoir located in Theni, Tamil Nadu. The inputs selected for the model included the following factors such as: ·Water level storage capacity ·Temperature ·Precipitation ·Wind speed ·Solar radiation ·Vapour pressure ·Inflow ·Outflow and ·Runoff. For this study, a dataset spanning twenty years was utilized, focusing on the Vaigai Reservoir located on the Vaigai River in Theni. A classification system based on performance indices, such as Root Mean Square Error (RMSE), Mean Square Error (MSE), Mean Absolute Error (MAE), and Mean Absolute Percentage Error (MAPE), was proposed and applied to identify the best-performing model. The A-FCNN combination achieved the highest accuracy across the datasets, with a predictive accuracy of 99.78% for the test data. Predicting sediment volume helps to improve water resource management, contributing to sustainable development and environmental protection.