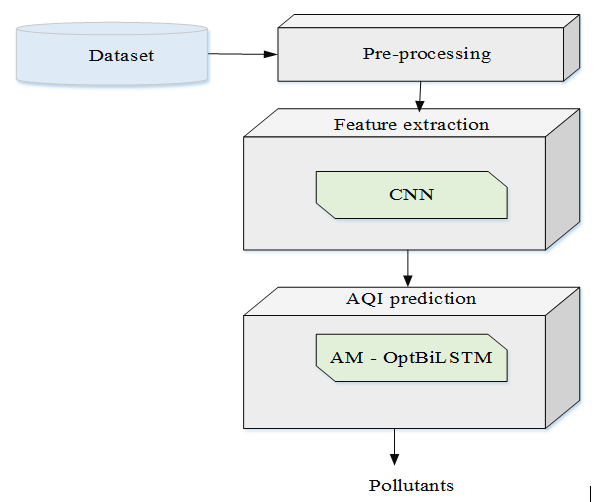
Predicting air pollution using environmental data assessment parameters becomes increasingly significant amid growing fears about climate change and the sustainability of urban areas. The use of sophisticated deep learning (DL) methods to model the intricate relation among these variables represents a promising area of research. However, current approaches have not effectively taken advantage of the temporal features derived from spatiotemporal correlations among air quality prediction systems, leading to poor long-term predictions. This work presents an AM (attention module) with CNN (convolutional neural network)-OptBiLSTM (optimal bidirectional long- and short-term memory) for AQIP (air quality index prediction). Here, the optimal process is carried out by the WSA (white shark algorithm). The analysis is demonstrated on the dataset and achieved better MSE and MAE values of 0.72 and 0.532 respectively. The developed model has the potential for application to other air pollutants. This proposed AM-CNN-OptBiLSTM has the capacity to substantially improve information services related to air quality prediction for the public. In addition, it provides support for regional pollution control and early warning systems.
Total file downloads: 23