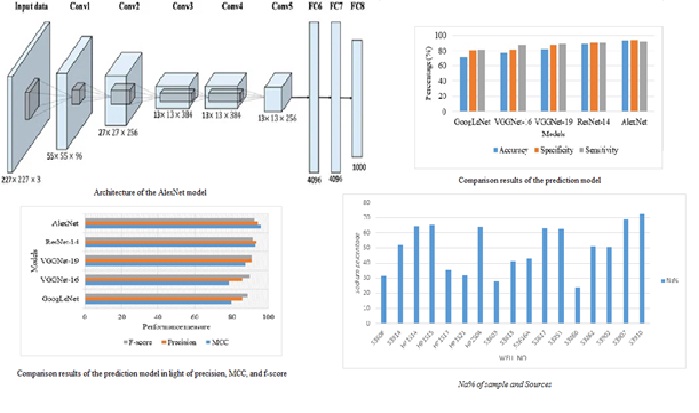
The purpose of this study is to anticipate and investigate the groundwater quality in the regions of the Cauvery basin. In the post-monsoon season of 2020, 800 samples were collected from 200 different places for this paper. The AlexNet model is used in this book to forecast the water quality. In order to examine the groundwater quality, the anticipated groundwater samples are analyzed using main cations (K+, Mg2+, Ca2+, and Na+), major anions (NO2- +NO3-, CO3-, F-, HCO3-, Cl-, and SO42-), hardness, pH, and Electrical Conductivity (EC). The accuracy, specificity, and sensitivity of the AlexNet model are used in this publication to validate its efficacy. Validating the following metrics determines if water is suitable for drinking and irrigation: sodium percentage, magnesium ratio, Kelly's ratio, sodium adsorption ratio, water quality index (WQI), and residual sodium carbonate (RSC). According to the research, the AlexNet model predicted water quality with 93%, 94.57%, and 91.47% accuracy, specificity, and sensitivity, respectively. According to the experimental results, the WQI value shows that 22% of the collected samples are acceptable for drinking, 63% are suitable for drinking, and the other 15% are only fit for irrigation and should not be consumed.