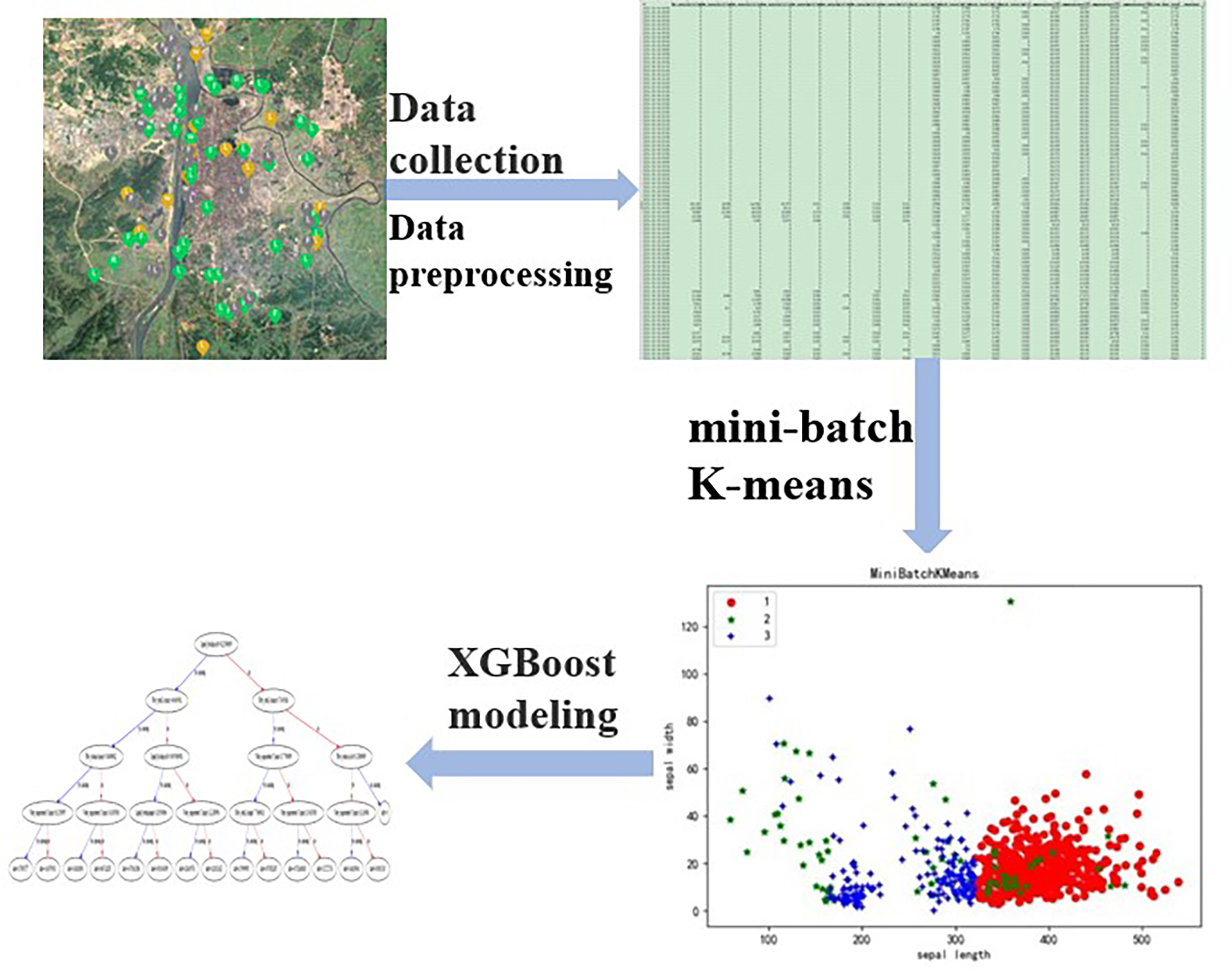
Identifying and classifying anomalies in on-line monitoring systems of drainage systems is important to reduce urban water pollution. In the context of big data, the mini-batch K-means combined with the XGBoost drainage network abnormal flow identification and classification model is proposed to precisely identify and classify abnormalities that occur in real-time updates of online drainage network data while resolving problems with subjectivity and the lack of uniform standards for classification. First, using Mini Batch K-means, the unclassified drainage network data were sorted into four categories: normal drainage, sneaky drainage, rainwater and sewage mixing, and inflow and infiltration. Next, XGBoost performs data modeling to create a model for classification and identification of drainage flow anomalies. To increase the accuracy of the model, the features were ultimately chosen based on the ranking of the importance of the features, and the model parameters were established using grid search and cross-validation. The results showed that the XGBoost Drainage Network Anomaly Classification and Identification Model can accurately identify four drainage network situations with high classification accuracy and good performance. It was also validated through the application of data from the online system monitoring points in Changsha, China, in 2020.
Total file downloads: 5