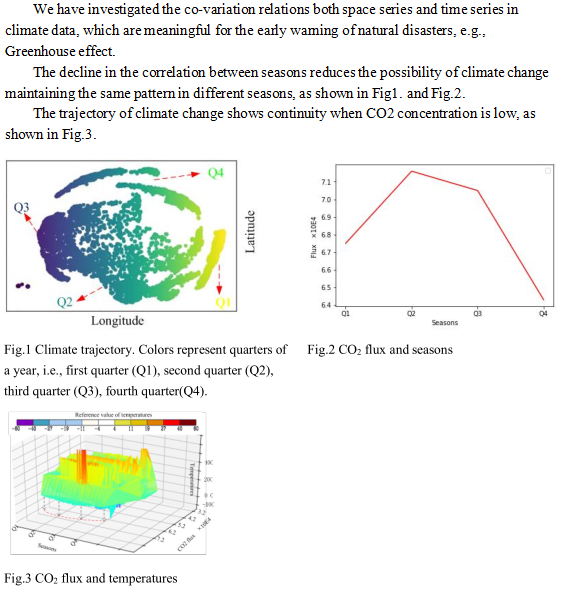
Climate data composes of time series and space series with unknown. These unknown series contains complex co-variation relations of climate data. The extraction of these relations is essential for further revealing the complex representations between time series and space series in climate data. As an important application, through extracting these co-variation relations, we can further predict the change of climate to provide early warning for natural disasters, e.g., Greenhouse effect. Hence, it is a challenge to explore the relations between climate data. To address this, this work propose a deep neural network. Based on Brenier theorem, the loss function is derived. Since Brenier theorem rigorously proves that the data distribution in background space is consistent with the data distribution in the feature space with greatest probability, ensuring that the relations extracted from the latent space are as close to that of in background space as possible. Then, the parameters of time series consisting of eight variables are encoded by the first hidden-layer in the proposed model. The remaining two hidden-layers encode the latitude and longitude in spatial series, respectively. Experimental results show that the proposed method outperforms the state-of-the-art methods with respect to climate relations extracted. Hence, the proposed method is considered a good alternative in capturing relations between climate variables, as well as, between carbon dioxide (CO2) and surface temperature
Total file downloads: 1