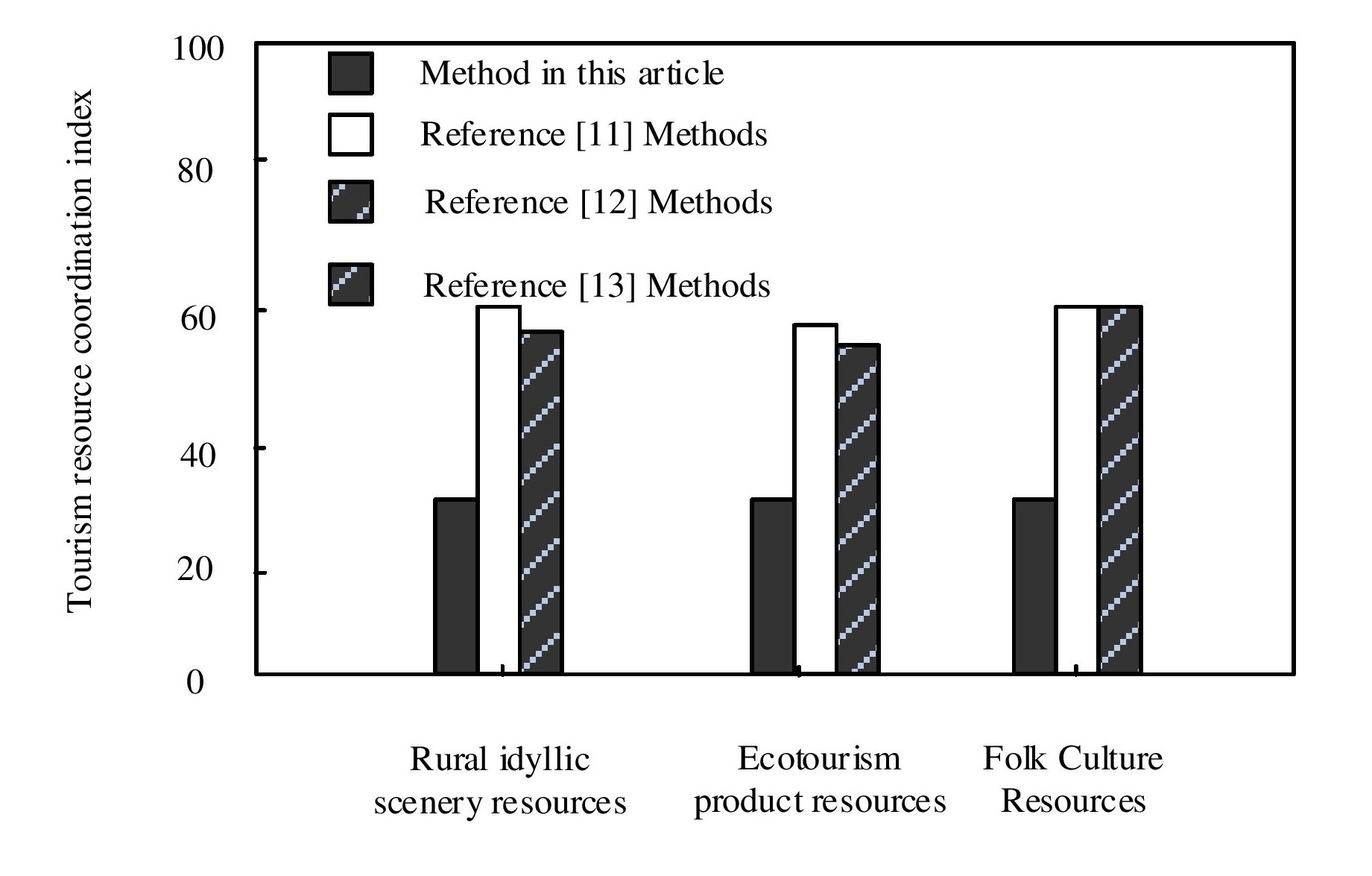
The development and layout of rural tourism resources is the core issue supporting the development and planning of tourism destinations. Currently, it mainly relies on the experience and judgment of planners, with strong subjectivity and lack of objective information technology support. Therefore, in the context of common prosperity, a method for distribution characteristics and development layout of rural tourism resource based on LSTM deep learning is proposed. After distinguishing various rural tourism resources using the classification method of rural tourism resources based on LSTM in-depth learning, the spatial distribution characteristics of various tourism resources are estimated using the analysis method for spatial distribution characteristics of tourism resources based on kernel density estimation; After identifying the current status of tourism resource functional areas through a method of tourism resource development and layout based on a grid spatial analysis model combined with the distribution characteristics information mastered, and from the perspective of tourists, it can optimize the layout mode of functional areas to maximize the benefits of rural tourism resources on the basis of satisfying tourists' preferences. After testing, this method can utilize intelligent information technology to optimize the development and layout of rural tourism resources.