- gnest_06890_proof.pdf
-
Paper IDgnest_06890
-
Paper statusProof
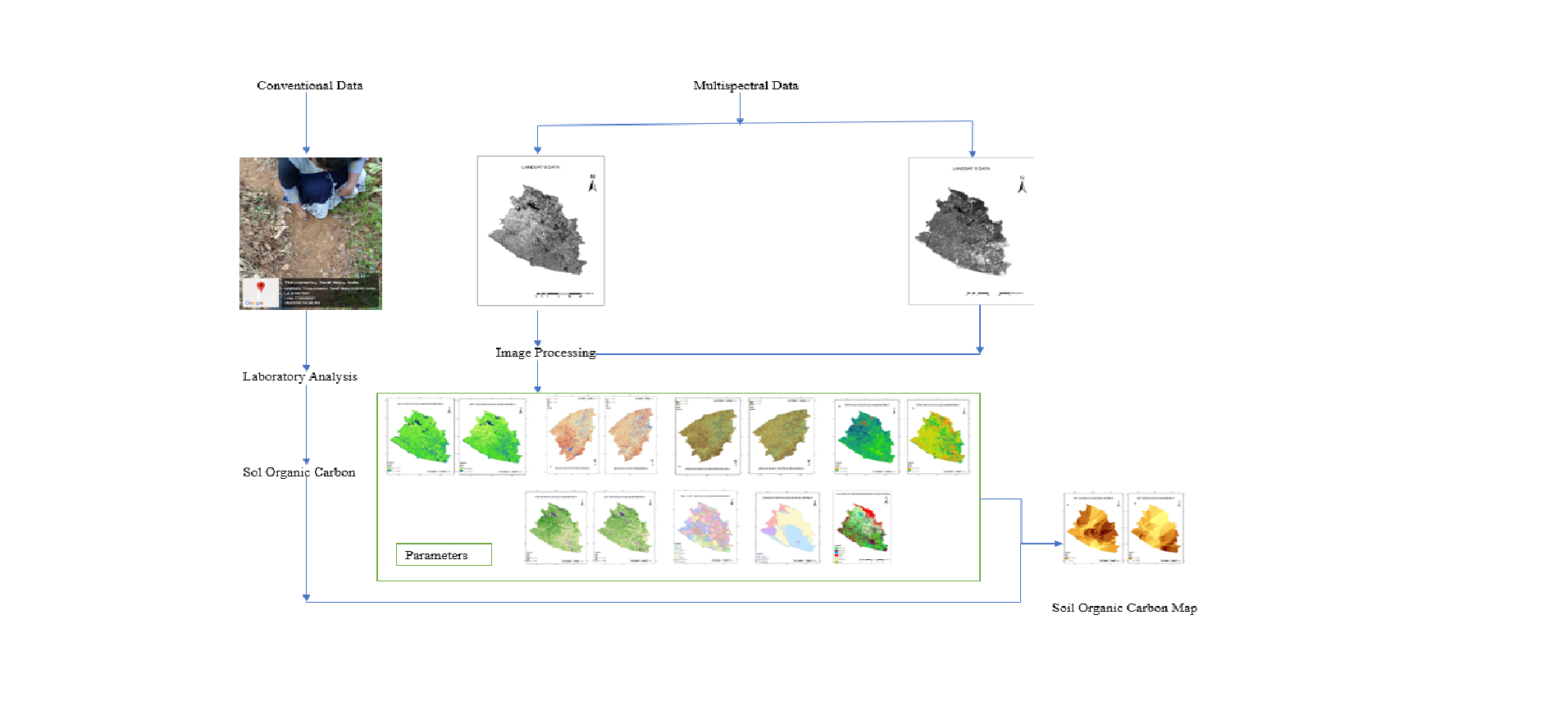
The Kanyakumari District was selected as a study area due to its diverse flora and favorable climate and soil conditions. Various remote sensing parameters were derived from Landsat 8 and 9 satellite data, including NDVI, BSI, NDMI, NDWI, and SAVI. Moreover, data on land use and land cover (LULC), geology, and soil type were considered. These parameters were utilized as inputs for Random Forest Regression Analysis to establish the relationship between Soil Organic Carbon (SOC) and each parameter, enabling predicting of future SOC levels. Comparing Landsat 8 and 9 data, BSI and NDWI displayed high correlations, while NDVI, NDMI, and SAVI exhibited medium correlations. To validate SOC predictions, 115 soil samples were collected from the field, and laboratory SOC content analysis was performed. Machine learning algorithms, specifically Random Forest Regression, were employed to predict SOC values. The predicted SOC values indicated spatial variations, with residential areas exhibiting low SOC and forested areas showing higher SOC due to minimal human disturbances. Creating a SOC map is instrumental in identifying regions requiring soil restoration and yield enhancement. This study underscores the utility of SOC mapping in guiding soil restoration efforts and enhancing agricultural productivity, with implications for precision farming and sustainable land management.
Total file downloads: 4