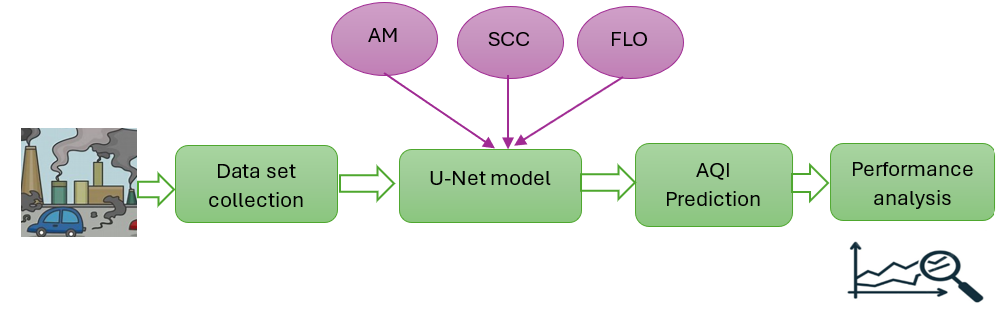
Air quality prediction is crucial for environmental monitoring and public health. This work presents a new prediction model for air quality prediction using a one-dimensional convolutional neural network (1D- CNN). The proposed model resembles U-Net architecture which is used in image segmentation tasks popularly. The proposed model uses the strength of U-Net’s encoder-decoder design to capture both local and global features effectively. To enhance the model's performance, we integrate attention mechanisms to focus on the most relevant features. In addition, the self-calibrated convolutions are applied to adjust the convolutional filters to improve feature representation. The parameters of the proposed model are fine-tuned using the Frilled Lizard Optimization (FLO) algorithm for optimal performance. Experimental results show that the proposed model significantly outperforms traditional methods in terms of validation loss and Mean Square Error rates.