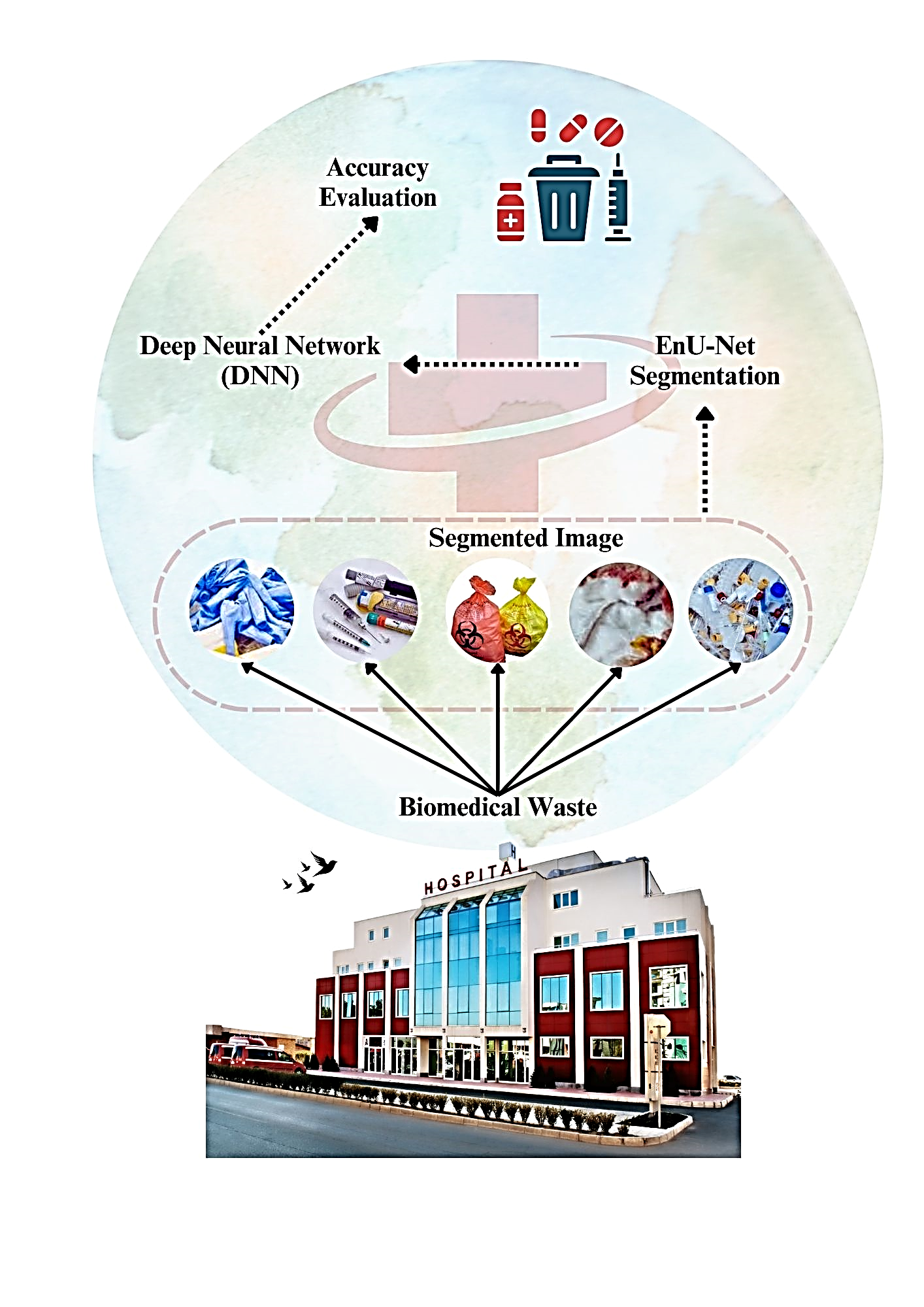
In India, biomedical waste (BMW) management is governed by strict regulations to mitigate health and environmental risks. It includes materials such as sharps, infectious wastes, pharmaceuticals, and non-hazardous items contaminated with potentially infectious substances. Proper management and disposal of BMW are critical to prevent environmental contamination and health risks. This article introduces an Enhanced U-Network (EnU-Net) integrated with Deep Neural Network BMW Classification (EnU-Net-DNN-BMWC) to enhance accuracy in this critical task. Leveraging the U-Net framework with an Encoder-Decoder Network (EDN) and pixel-wise classification layer initially optimizes image segmentation. Bayesian functions mitigate segmentation uncertainties, while Content-Sensitive Sampling (CSS) refines pixel sampling to prioritize data-sparse regions. Data collected from G. Kuppuswamy Naidu Memorial Hospital and Kovai Medical Center and Hospital (KMCH) in Coimbatore over six months, categorizing sharps, infectious materials, pharmaceuticals, and non-hazardous waste, informs waste management strategies. Experimental validation using 100 biomedical waste images demonstrates EnU-Net-DNN-BMWC achieving good accuracy, surpassing standalone DNN-BMWC using Matlab 2022. The comparative analysis across different metrics such as accuracy, precision, F-measure, recall, error rate, and RMSE between DNN-BMWC and the proposed EnU-Net-DNN-BMWC framework were evaluated in this study, highlighting EnU-Net-DNN-BMWC's superior performance. Finally, this study underscores EnU-Net-DNN-BMWC’s efficacy in enhancing biomedical waste classification, crucial for sustainable waste management practices and regulatory compliance in healthcare settings.
Total file downloads: 25