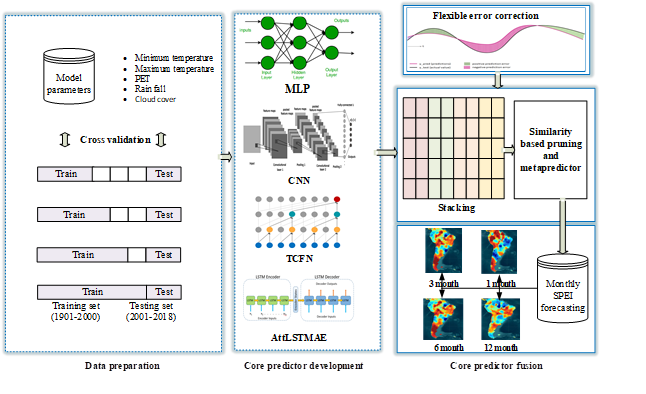
Investigating the influence of climate change on drought in a dynamic environment is essential for human society, agriculture, and ecology. Draught forecasting often considers machine learning techniques that use climate-mode indices as predictor variables. However, forecasting in long lead times is still difficult due to the consequences of climate change and the difficulties associated with evaluating drought. In this study, a novel ensemble learning with optimized pruning model (EnsLOP) based on different deep learning models, i.e., multilayer perceptron (MLP), convolutional neural network (CNN), temporal convolutional feature network (TCFN) and Attention-driven LSTM Autoencoder (AttLSTMAE), is applied to improve the forecasting capability of draught index namely Standardised Precipitation Evapotranspiration Index (SPEI). This study collects the preceding lag memory of climatic mode indicators such as rainfall, temperature, precipitation, and cloud cover as predictor variables to achieve significantly accurate draught forecasts. Also, a new flexible error correction (FEC) is proposed to reduce the prediction errors of the core predictors. The simulation results demonstrate that the proposed EnsLOP model gained a distinct advantage in terms of SPEI prediction with comparatively low relative errors (RMSE =0.098 and R2 <0.098).