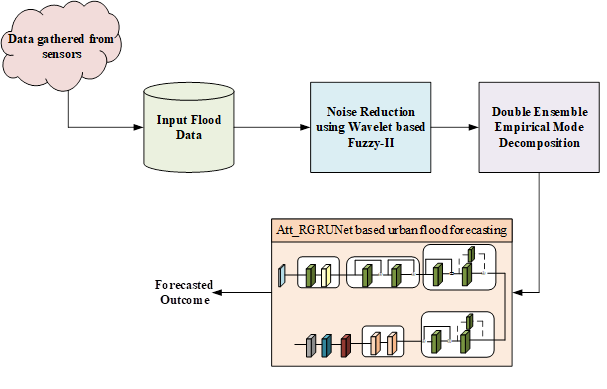
Flood forecasting is significant for hydrology and disaster management due to the complex and nonlinear nature of flood-related data. There are several conventional forecasting methods often failed to effectively capture both spatial and temporal dependencies that leads to inaccurate predictions. In order to overcome the challenges, a novel method is introduced with data de-noising and deep learning models for flood prediction. The proposed approach comprises of three key steps, wherein de-noising of flood data is employed initially using wavelet transform with Fuzzy II threshold selection to eliminate noise in capturing significant features. Then, Intrinsic Mode Function (IMF) extraction is employed using Double Ensemble Empirical Mode Decomposition (DEEMD) to acquire appropriate flood patterns. Finally, flood forecasting is employed using an Attentive Residual Gated Recurrent Unit (Att_RGRUNet) model, wherein ResNet is utilized extracts spatial features, GRU model is utilized for temporal dependencies, and coordinate attention mechanism for enhancing the feature representation. The combined approach ensures high predictive accuracy and enhances early warning systems. The proposed model is evaluated based on RMSE, MAE and MAPE and acquired the values of 0.76984, 0.8 and 2.94 respectively.
Total file downloads: 15