-
Thematic area
-
Topic
-
VolumeVolume 27 (2025)
-
Issue
-
Pages1-12
- gnest_06937_final.pdf
-
Paper IDgnest_06937
-
Paper statusPublished
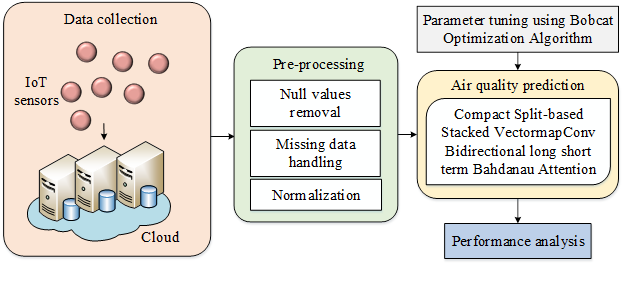
Air pollution is one of the serious environmental problem that has an impact on ecosystems and human health worldwide. The prediction of air pollution can provide significant information that will permit all parties to take right initiatives. Predicting air quality is considered as a popular research area. A potential solution to air pollution has been suggested through the application of numerous time-series and artificial intelligence (AI) methods. These models are used with Internet of Things (IoT) devices in a cloud environment to forecast the air quality parameters. However, there exist several challenges such as overfitting issues, inaccurate real-time updates, and low precision. In this paper, an IoT cloud-based Air Pollution Monitoring System using Deep Learning (IoT-CAPM-DL) model under various meteorological situations is proposed to address the existing problems. Data collected from sensors are preprocessed to enhance its quality by eliminating null values, handling missing data, and normalization. Then, a robust Compact Split-based Stacked VectormapConv Bidirectional long short term Bahdanau Attention (CSplitStack-VBA) network is used to predict air quality parameters. A Bobcat Optimization Algorithm (BcOA) is used to tune the hyper-parameters of prediction model. The entire implementation is carried out using the Python platform and different kind of performance measures are calculated. The finding shows that the IoT-CAPM-DL model attains better MAE and RMSE value of 0.0076 and 0.0051. Thus, the experimental outcomes prove that the IoT-CAPM-DL model performed better in the prescribed dataset and produced significant results than existing approaches.