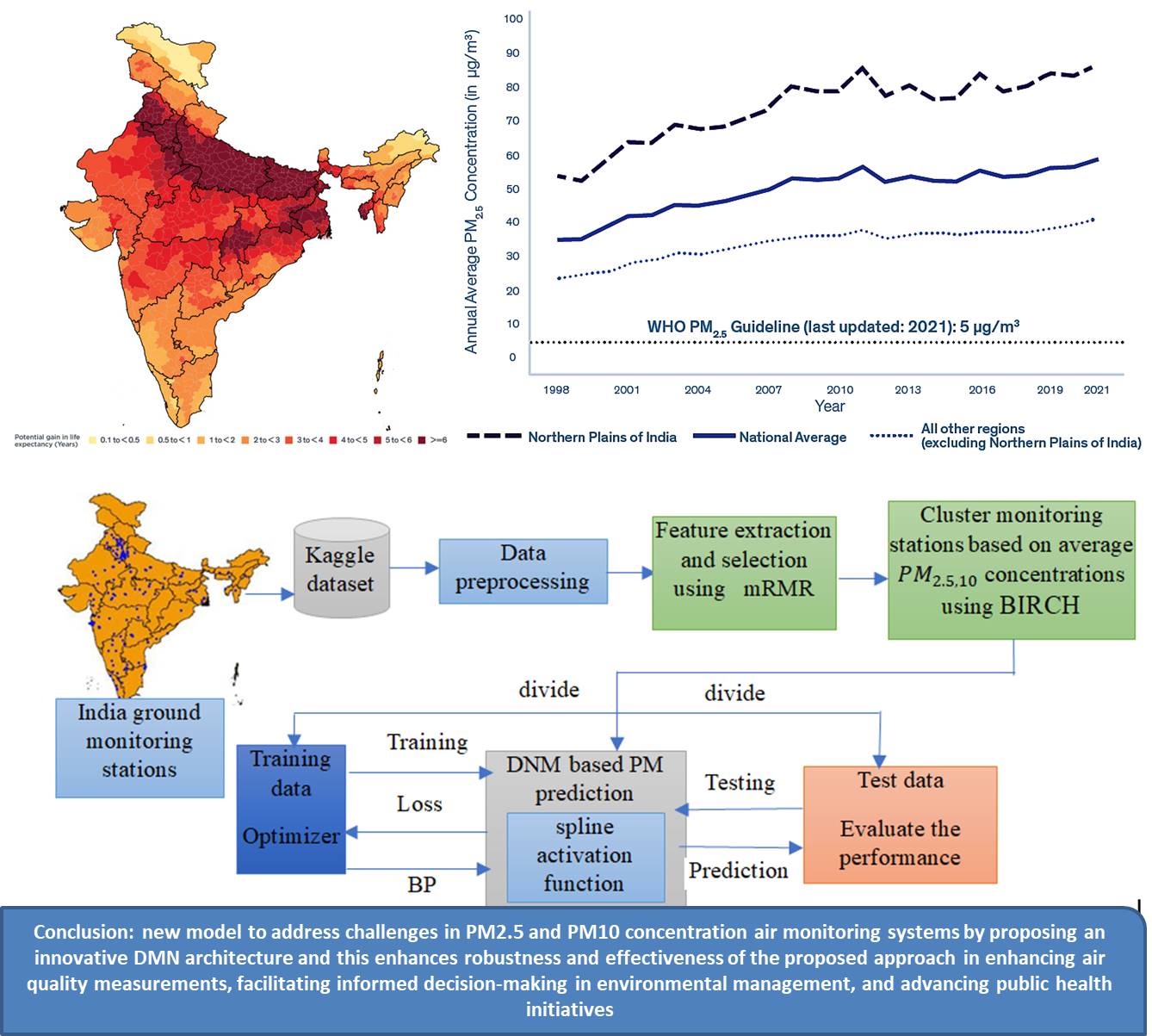
Currently, most of the global population resides in metropolitan areas, where air quality standards are not properly monitored. As a result, people are constantly exposed to air contaminants that exceed the thresholds set by the World Health Organization (WHO). Air quality monitoring system is often encountering challenges such as discontinuities and missing data in time sequences, affecting the accuracy of measurements. This paper presents an innovative approach to address these issues in PM 2.5 and 10 concentration air monitoring systems proposes a novel Deep Maxout Network (DMN) architecture enhanced with Polynomial and Spline Interpolation methods to effectively handle the discontinuities in data sequences. By smoothing transition fitting curves at interval junctions, the proposed model generates an ideal dataset, improving the robustness and accuracy of air quality measurements. First, the data is collected and pre-processed. Then, the features are extracted and selected by using minimum redundancy maximum relevance (mRMR). Then similar features are clustered by using Balanced Iterative Reducing and Clustering using Hierarchies (BIRCH) scheme. Finally, the PM concentration is predicted by using DMN. Experimental results demonstrate the effectiveness of the proposed approach in enhancing the reliability of Matter2.5 and 10 concentration monitoring systems using Air Quality Data in India from kaggle, offering a promising avenue for precise P M 2.5 and 10 concentration forecasting with practical implications for air quality management and public health initiatives.
Total file downloads: 12