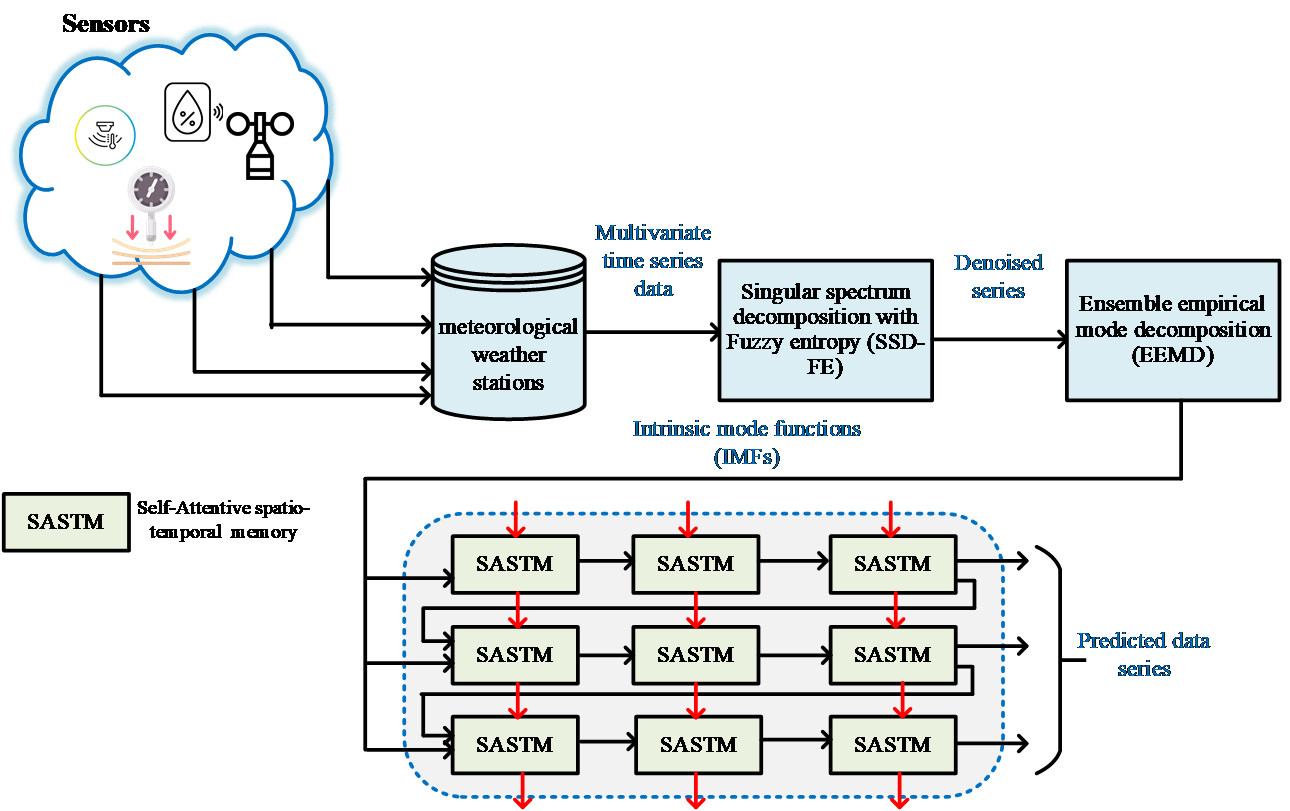
Weather forecasting is an essential but complex task because of its substantial influence on human life and advanced atmospheric dynamics. In recent years, deep learning-based techniques have gain high attention for weather forecasting. Nevertheless, current forecasting models ignore the interdependent relationship between variables across regions and primarily examine temporal patterns of weather data. In this work, a new Multivariate time series weather forecasting model is proposed using integrated secondary decomposition and Self-Attentive spatio-temporal learning network (SASTLNet). Particularly, the weather data is filtered using a Singular Spectrum Decomposition with Fuzzy Entropy (SSD-FE) for removing the irrelevant components and screening the component containing vital information. Moreover, the spatiotemporal fluctuations in the meteorological variables are investigated using an enhanced empirical mode decomposition (E3MD) method and an SASTLNet model. The self-attention block of the SASTLNet model is discovered using ladder format to lower the processing costs while representing the temporal and spatial features through single memory cell. The simulation results prove that the suggested model can outperforms the baseline models in terms of efficacy and accuracy.
Total file downloads: 12