-
Thematic area
-
Topic
- gnest_06192_in press.pdf
-
Paper IDgnest_06192
-
Paper statusIn press
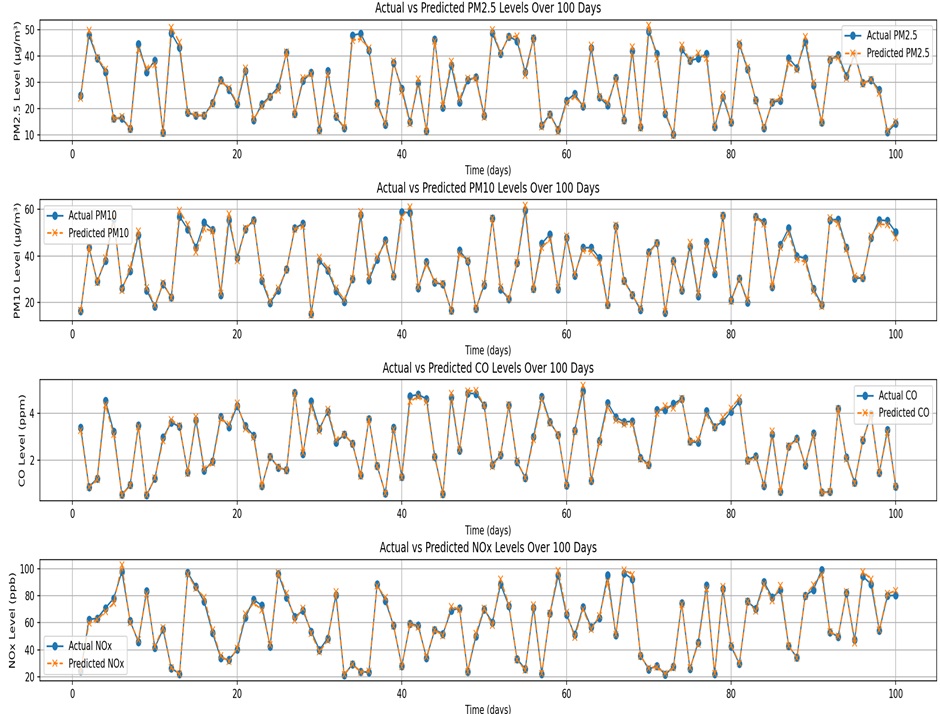
In recent days air quality has been very poor in urban society due to a lot of industries, vehicles and so on. To overcome poor quality, an air quality prediction is much needed to manage an urban area and control pollution. In this article, deep learning (DL) namely a Modified Gated Recurrent Unit (GRU) neural network is used to forecast air pollution. Initially, a new activation function named Dual-Slope Leaky ReLU (DSL-ReLU) is introduced. The DSL-ReLU activation function includes two hyperparameters which control the slopes for positive and negative input values to achieve fine-tuned responses for varying data inputs. Then, the model parameters like learning rate, batch size, and dropout rates are tuned using the Spider Wasp Optimization (SWO) algorithm. The SWO algorithm is based on the female spider wasp’s hunting and nesting behaviour that is applied to solve the optimization issues. This SWO model is used to GRU model parameters and provides robustness in the predictive modelling of air quality monitoring. For validation, the Kaggle data set is used whereas the proposed optimized GRU model considerably improves the accuracy of air pollution forecasts