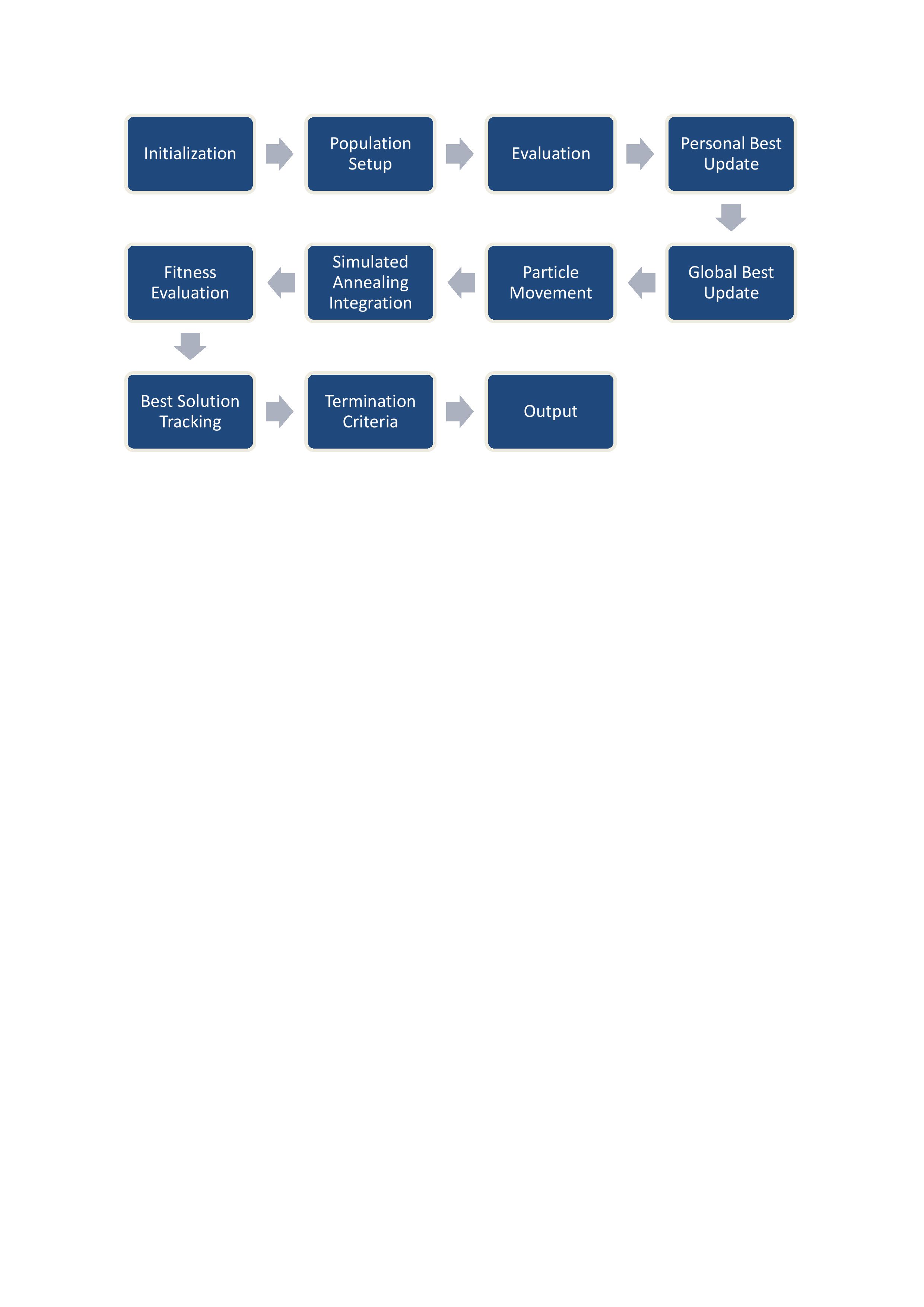
Gene expression data analysis is crucial for understanding complex biological mechanisms, yet current biclustering techniques struggle with noise, unpredictability, and intrinsic uncertainty. This study proposes an innovative biclustering method combining particle swarm optimization (PSO), simulated annealing (SA), and fuzzy logic to improve gene expression analysis. By integrating natural language processing (NLP) semantic similarity into the PSO framework, the method enhances the capture of intricate gene interactions. Additionally, environmental factors, particularly air pollution, are incorporated to explore their impact on gene expression patterns. The approach leverages the complementary strengths of PSO's exploration capabilities, SA's exploitation efficiency, and fuzzy logic's ability to handle data ambiguity. Comparative assessments on benchmark datasets reveal that this integrated strategy significantly outperforms individual methods in accuracy and resilience. The results demonstrate the PSO-SA-Fuzzy Logic method's superior capacity to detect nuanced and context-dependent gene expression patterns, offering a robust solution to the limitations of existing biclustering techniques. The method not only improves precision and computational efficiency but also enhances the detection of significant gene expression patterns under varying conditions, including environmental stressors. This advancement represents a notable contribution to computational biology, providing a more effective tool for gene expression data analysis.