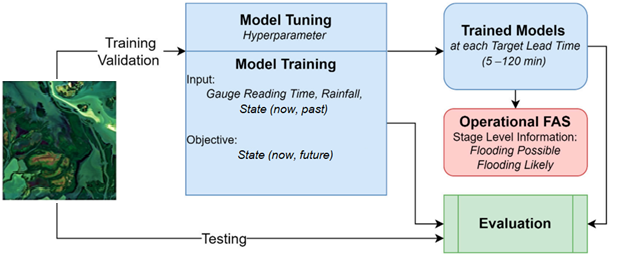
Urban flooding has severely threatened the ecosystem and human life in recent years. The key to managing stormwater is to understand what causes it. The forceful effects of building shape on urban floods should be addressed, which results in a significant underestimation of flood danger. Algorithms for data-driven machine learning shed light on how the placement of buildings affects urban flooding. This study aimed to identify the elements of flooding risk and their effects on nearby communities using a concatenated modelling loop that included the XGBoost algorithm. This work suggests an enhanced extreme gradient boosting (XGBoost) approach based on a concatenated boosting particle swarm optimization (CBPSO) operator to acquire the meteorological refractive index of 100 m over the ocean. The prediction results of the enhanced XGBoost algorithm are compared with those of the backpropagation (BP) network and the original XGBoost method using the evaluation criteria Accuracy, Precision, Recall, F1-score, and IoU.Moreover, the networks resolve the featured misaligned issue during the decoder by inserting a component synchronization module into the up-sampling procedure. The model's intersections of unions (IoU) of 89.90% outperformed SOTA flood detection systems.