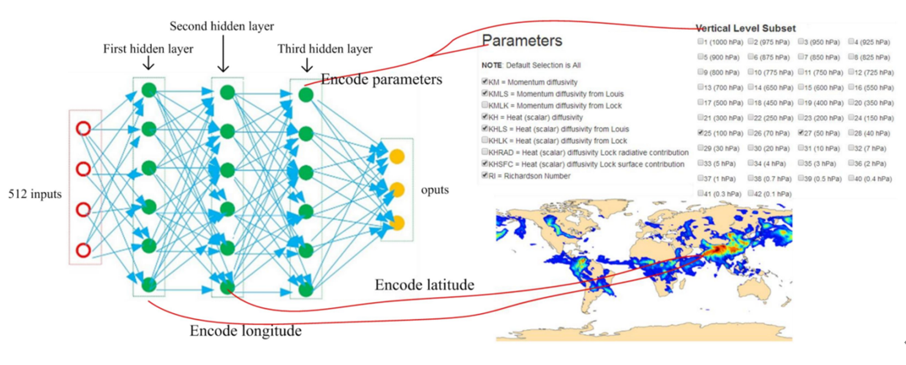
Climate data contains rich information and show high dimensions. Through exploring the data trajectory of climate data, climate change can be forecasted. Climate data is typical high-dimensional data, unlike shallow structures, deep structures have more advantages in handling high-dimensional data. Deep neural networks with multiple layers have ability of the data trajectory discovery from the complicated high-dimensional data. Here, this paper seeks to explore the data trajectory from complicated climate data using the proposed deep neural networks. First, using the sampling theorem to reconstruct the discrete surface that can converge to the original space. Thereafter, to minimize the error between the original data trajectory and the extracted data trajectory, the Brenier theoretical function in Brenier Theorem is used as the loss function of the deep neural networks. By doing so, the deep neural networks can extract the data trajectory from climate data as accurate as possible. Finally, as an important application, since a thorough understanding of the data trajectory of climate data is essential for understanding the effect of earth’s future climate.