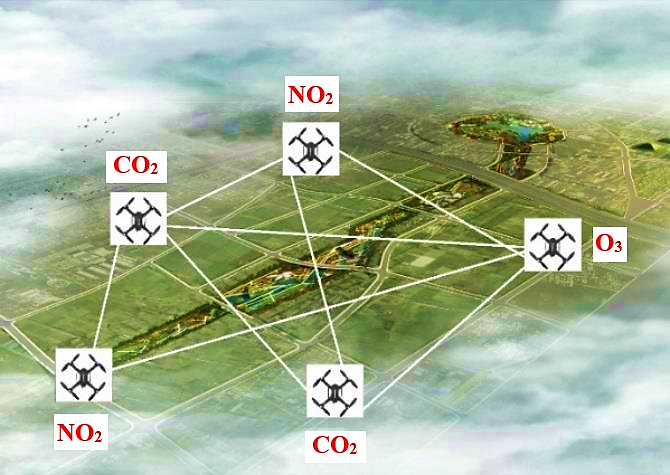
Air pollution poses significant threats to ecosystems, atmosphere, and the health of both humans and wildlife. It is primarily caused by industrial and chemical pollutants, leading to deterioration in air, water, and soil quality. As a result, effective air quality monitoring is crucial for environmental management. Flying Mobile Ad hoc Networks (FMANETs) offer a promising solution for intelligent air quality monitoring and assessment by employing unmanned aerial vehicles (UAVs) to measure air pollutants. However, FMANET-based systems face unique challenges, including UAVs' three-dimensional movement, high dynamism, rapid topological changes, resource constraints, and low UAV density. Therefore, routing optimization becomes a fundamental issue in these systems. In this paper, we propose a novel routing method named RFMAN (Reward-learning-based FMANET) for intelligent air quality monitoring systems. RFMAN consists of two main components: route discovery and route maintenance. In the route discovery phase, we introduce a Reward-learning-based mechanism to identify optimal routes, while also implementing a filtering parameter to refine the UAV selection process and reduce the search space. The route maintenance phase of RFMAN focuses on detecting and correcting paths nearing breakdowns, as well as promptly replacing failed paths to ensure continuous monitoring efficiency. The result section pictures that RFMAN outperforms other methods. Overall, RFMAN offers a promising solution for enhancing the effectiveness and reliability of air quality monitoring in FMANET-based systems.
Total file downloads: 31