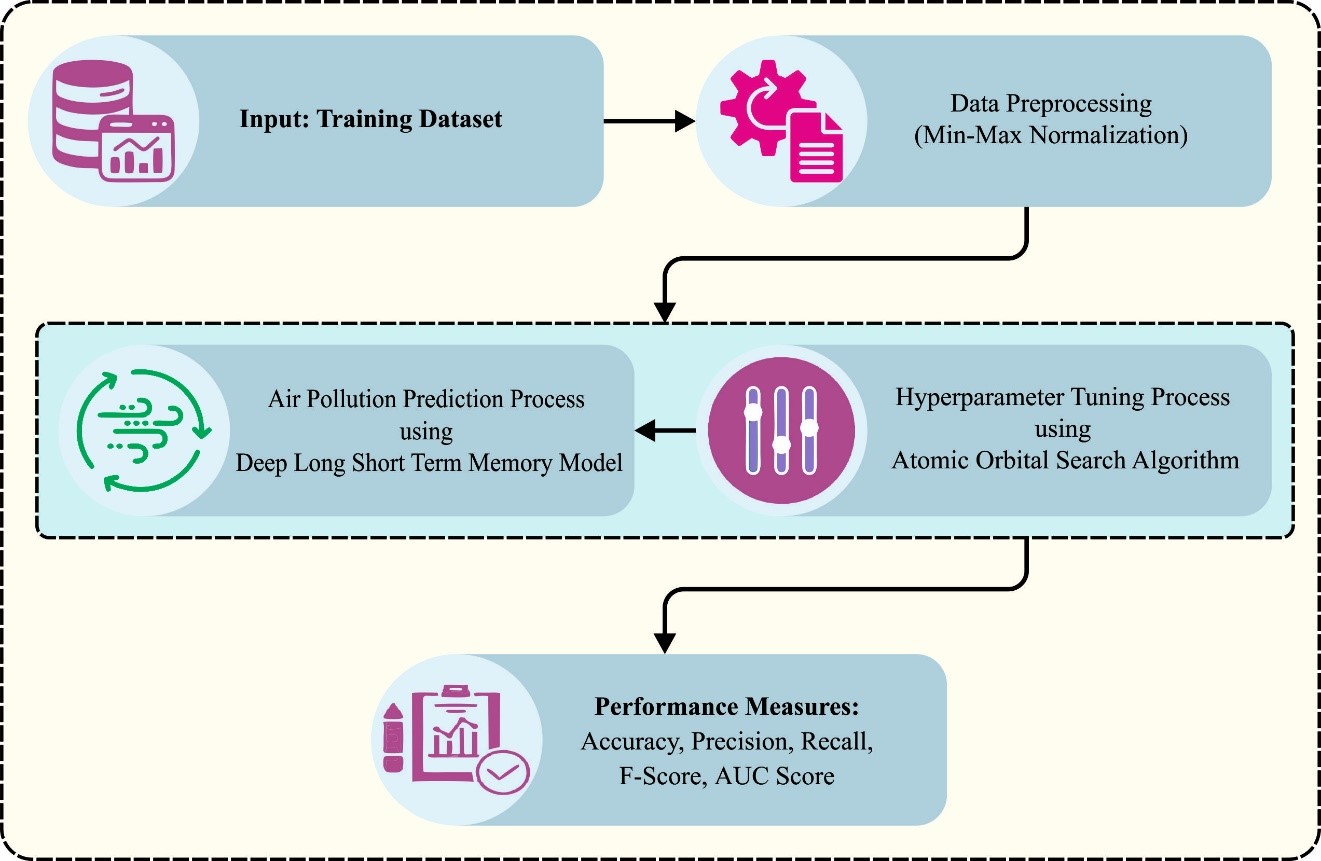
Air pollution is a major reason for health-related issues and weather changes, one of humanity's most dangerous problems. It is the most crucial environmental issue in the 21st century and has attracted global attention. These challenges are exacerbated by an overabundance of automobiles, industrial output pollution, transportation fuel consumption, and energy generation. Therefore, air pollution prediction was developed vital. Air pollution monitoring is the procedure of analyzing and measuring the air quality in a certain place to develop the levels of several pollutants and contaminants present. Monitoring air pollution is vital to understand its sources, and effects on the environment and human health, and for executing methods for mitigating its effects. Deep learning (DL) approaches are employed for air pollution predicting methods. Therefore, this study develops an Atomic Orbital Search Algorithm with a Deep Learning-Driven Air Pollution Monitoring (AOSADL-APM) approach. The purpose of the AOSADL-APM technique is to predict and classify the presence of air pollutants. In the presented AOSADL-APM technique, the min-max normalization approach is applied for data pre-processing. For air pollution prediction and classification, the AOSADL-APM technique applies deep long short-term memory (DLSTM) methodology. To enhance the performance of the AOSADL-APM technique, the AOSA-based hyperparameter tuning has been developed. The simulation results of the AOSADL-APM technique were tested using the benchmark dataset. The widespread outcome analyzed the greater solution of the AOSADL-APM algorithm compared to existing approaches.
Total file downloads: 10